A Real-time Semantic Segmentation Approach with Gated Multilayer Fusion
A multi-layer fusion and semantic segmentation technology, applied in the field of computer vision, can solve problems such as loss and large effective features, and achieve the effect of improving restoration, increasing running speed, and promoting feedback and supervision
- Summary
- Abstract
- Description
- Claims
- Application Information
AI Technical Summary
Problems solved by technology
Method used
Image
Examples
Embodiment Construction
[0026] In order to make the object, technical solution and advantages of the present invention clearer, the present invention will be further described in detail below in conjunction with specific examples.
[0027] A real-time semantic segmentation method of gated multi-layer fusion, specifically including the following steps:
[0028] (1) Construct a gated multi-layer fusion network:
[0029] The gated multi-layer fusion network for semantic segmentation constructed by the present invention, such as figure 1As shown, including 64-dimensional 1 / 2 times downsampling layer, 128-dimensional 1 / 4 times downsampling module, 256-dimensional 1 / 8 times downsampling module, 512-dimensional 1 / 16 times downsampling module, 1028-dimensional 1 / 32 times downsampling module of 512 dimensions, 2 times upsampling module of 512 dimensions, 2 times upsampling module of 256 dimensions, 4 times upsampling module of 256 dimensions, 2 times upsampling module of 128 dimensions, 4 times of 128 dimens...
PUM
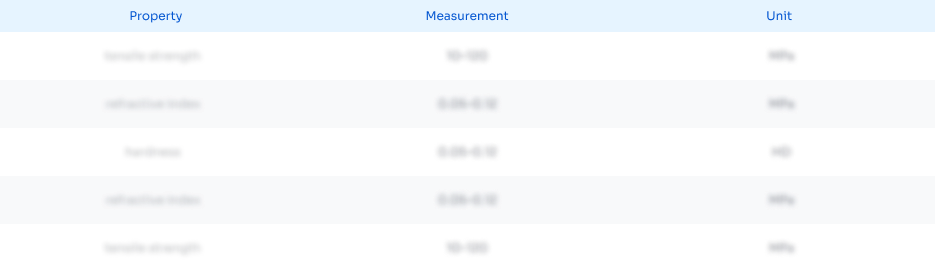
Abstract
Description
Claims
Application Information
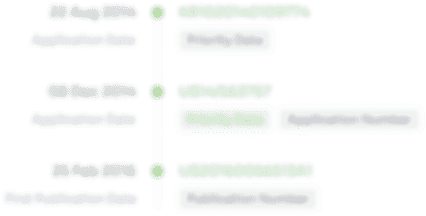
- R&D Engineer
- R&D Manager
- IP Professional
- Industry Leading Data Capabilities
- Powerful AI technology
- Patent DNA Extraction
Browse by: Latest US Patents, China's latest patents, Technical Efficacy Thesaurus, Application Domain, Technology Topic, Popular Technical Reports.
© 2024 PatSnap. All rights reserved.Legal|Privacy policy|Modern Slavery Act Transparency Statement|Sitemap|About US| Contact US: help@patsnap.com