Sample screening and expression recognition method, neural network, equipment and storage medium
A screening method and neural network technology, applied in the computer field, can solve the problem that the accuracy of expression recognition cannot be effectively improved, and achieve the effect of improving the accuracy of recognition
- Summary
- Abstract
- Description
- Claims
- Application Information
AI Technical Summary
Problems solved by technology
Method used
Image
Examples
Embodiment 1
[0029] figure 1 The implementation process of the sample screening method provided by the first embodiment of the present invention is shown. For the convenience of description, only the parts related to the embodiment of the present invention are shown, and the details are as follows:
[0030] In step S101, a multi-group sample is obtained, and the multi-group sample includes: an anchor sample, a positive sample and a negative sample.
[0031] In this embodiment, the multi-tuple samples may be triple samples, quadruple samples, and the like. Taking triplet samples as an example, triplet samples include: anchor samples (also called fixed samples or benchmark samples), positive samples similar to anchor samples, and negative samples not similar to anchor samples. Taking the quadruple sample as an example, the quadruple sample includes: an anchor sample, a positive sample similar to the anchor sample, a first negative sample dissimilar to the anchor sample, and a second negativ...
Embodiment 2
[0039] On the basis of Embodiment 1, this embodiment further provides the following content:
[0040] In this embodiment, the first sample distance and the second sample distance are random sample distances and obey the normal distribution, and according to the distribution statistical characteristics of the first sample distance and the second sample distance, construct Boundary conditions for screening the multigroup samples, specifically including as figure 2 The flow shown:
[0041] In step S201, using the modulus of the multigroup sample in the fully connected layer in the neural network, and the feature dimension of the multigroup sample in the fully connected layer, determine the mean and standard deviation of the normal distribution;
[0042] In step S202, using the mean value, the standard deviation, the first significance level corresponding to the positive sample, and the second significance level corresponding to the negative sample, construct a first A sub-bounda...
Embodiment 3
[0047] This embodiment provides a method for facial expression recognition, including:
[0048] The image to be recognized is processed by using the neural network obtained through the training of the sample selection method in the above-mentioned embodiments, and the facial expression recognition result is obtained.
[0049] In order to further improve the accuracy of calculation processing, in other embodiments, the expression recognition method further includes: performing side face screening on the multi-group samples, and / or performing occlusion screening on the multi-group samples. Through side face screening and / or occlusion screening, the retention results obtained from the screening can be further processed by the above-mentioned sample screening method.
PUM
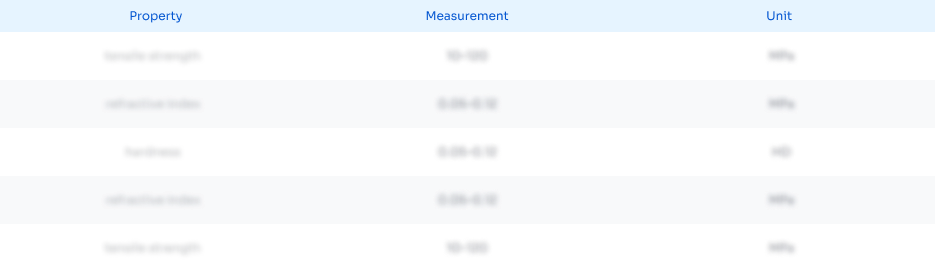
Abstract
Description
Claims
Application Information
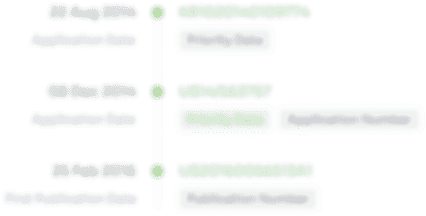
- R&D Engineer
- R&D Manager
- IP Professional
- Industry Leading Data Capabilities
- Powerful AI technology
- Patent DNA Extraction
Browse by: Latest US Patents, China's latest patents, Technical Efficacy Thesaurus, Application Domain, Technology Topic, Popular Technical Reports.
© 2024 PatSnap. All rights reserved.Legal|Privacy policy|Modern Slavery Act Transparency Statement|Sitemap|About US| Contact US: help@patsnap.com