SD-OCT denoising method based on unsupervised adversarial neural network
A SD-OCT, EDI-OCT technology, applied in neural learning methods, biological neural network models, image data processing, etc., can solve the problems of a large number of label samples, poor SD-OCT denoising effect, etc., to remove image noise. and the effect of bar artifacts
- Summary
- Abstract
- Description
- Claims
- Application Information
AI Technical Summary
Problems solved by technology
Method used
Image
Examples
Embodiment Construction
[0032] The present invention will be further described below in conjunction with the accompanying drawings and embodiments.
[0033] The present invention comprises the steps:
[0034] Step 1: Collect N SD-OCT images and M EDI-OCT images, M EDI-OCT images are the denoising images of M SD-OCT images respectively, N>M; extract N SD-OCT images respectively Retinal anatomical structure area, register the EDI-OCT image with its corresponding SD-OCT image, and find the retinal anatomical structure area in the EDI-OCT image;
[0035] Step 2: Take the retinal anatomical structure area in N SD-OCT images as image data samples, and M EDI-OCT images as sample labels to construct an image data sample set;
[0036] Step 3: Design a recurrent generative confrontation network with global structure and local structure constraints;
[0037] Step 4: Use the image data sample set to train the recurrent generative confrontation network to obtain the SD-OCT denoising model sensitive to structura...
PUM
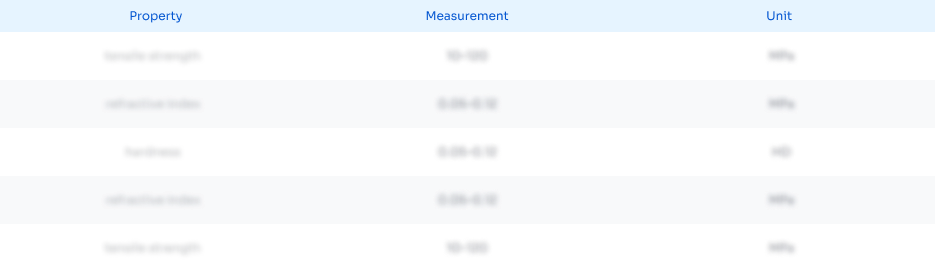
Abstract
Description
Claims
Application Information
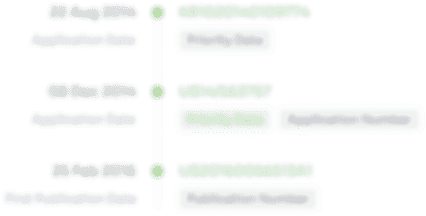
- R&D Engineer
- R&D Manager
- IP Professional
- Industry Leading Data Capabilities
- Powerful AI technology
- Patent DNA Extraction
Browse by: Latest US Patents, China's latest patents, Technical Efficacy Thesaurus, Application Domain, Technology Topic, Popular Technical Reports.
© 2024 PatSnap. All rights reserved.Legal|Privacy policy|Modern Slavery Act Transparency Statement|Sitemap|About US| Contact US: help@patsnap.com