Rolling bearing fault classification method based on mixed feature extraction
A rolling bearing and hybrid feature technology, applied in the field of rolling bearing fault classification based on hybrid feature extraction, can solve the problems of unavoidable errors and unknown interference, time-consuming and laborious, insufficient representation ability, etc., so as to reduce the computational complexity and accuracy of fault classification. interference, reasonable selection, and the effect of improving classification accuracy
- Summary
- Abstract
- Description
- Claims
- Application Information
AI Technical Summary
Problems solved by technology
Method used
Image
Examples
Embodiment Construction
[0040] The present invention will be described in further detail below in conjunction with the accompanying drawings.
[0041] refer to figure 1 , a rolling bearing fault classification method based on hybrid feature extraction, including the following steps:
[0042] A. Collect acoustic emission signals of rolling bearings under different working conditions, perform feature extraction on them, and construct mixed features;
[0043] The high-dimensional mixed feature vector of the present invention is formed original feature set F=(f by 21 features 1 , f 2 ,..., f 21), including 5 waveform features extracted by the waveform feature parameter method and 10 time-domain and 6 frequency-domain features extracted by the waveform analysis method. Waveform characteristics have a rise time (f 1 ), count (f 2 ), duration (f 3 ), magnitude (f 4 ) and energy (f 5 ); time-domain statistical features have a mean (f 6 ), RMS value (f 7 ), peak (f 8 ), square root magnitude (f 9...
PUM
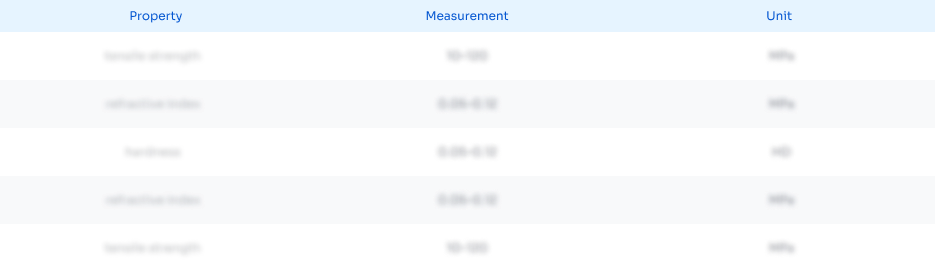
Abstract
Description
Claims
Application Information
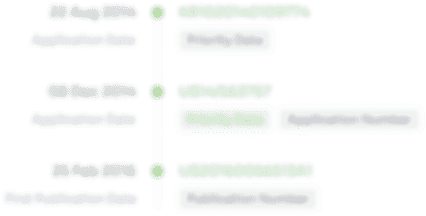
- R&D Engineer
- R&D Manager
- IP Professional
- Industry Leading Data Capabilities
- Powerful AI technology
- Patent DNA Extraction
Browse by: Latest US Patents, China's latest patents, Technical Efficacy Thesaurus, Application Domain, Technology Topic.
© 2024 PatSnap. All rights reserved.Legal|Privacy policy|Modern Slavery Act Transparency Statement|Sitemap