Human body characteristic parameter prediction method based on semi-supervised learning
A semi-supervised learning and human body feature technology, applied in the field of human body feature parameter prediction based on semi-supervised learning, can solve the problems of non-existence, reduced acquisition cost, and large construction workload, so as to reduce the requirements of training samples and reduce data collection. cost, the effect of reducing data volume requirements
- Summary
- Abstract
- Description
- Claims
- Application Information
AI Technical Summary
Problems solved by technology
Method used
Image
Examples
Embodiment Construction
[0048] In order to make the purpose, technical solution and advantages of the present invention clearer, the present invention will be further described in detail and clearly below in conjunction with the accompanying drawings and embodiments.
[0049] figure 1 Be the schematic diagram of the steps of the inventive method, the specific embodiment of the present method and implementation steps are as follows:
[0050] S1. Build a data set, including a labeled data set based on a real human body and an unlabeled data set based on a virtual human body;
[0051] S101, such as figure 2 As shown in Fig. 1, collect frontal images of real human body with different arm postures in a standing posture. The subjects are required to stand on a level ground with their feet shoulder-width apart, and keep their arms in the same plane as the human torso. Collect 3-4 groups of different arm postures. and other data;
[0052] S102, such as image 3 As shown in , the frontal images of the rea...
PUM
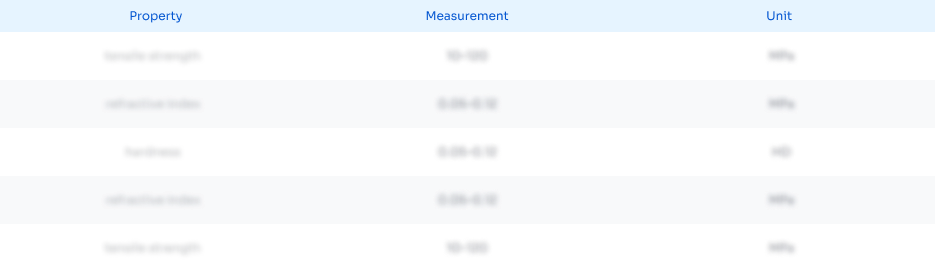
Abstract
Description
Claims
Application Information
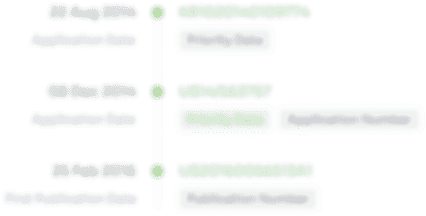
- R&D Engineer
- R&D Manager
- IP Professional
- Industry Leading Data Capabilities
- Powerful AI technology
- Patent DNA Extraction
Browse by: Latest US Patents, China's latest patents, Technical Efficacy Thesaurus, Application Domain, Technology Topic, Popular Technical Reports.
© 2024 PatSnap. All rights reserved.Legal|Privacy policy|Modern Slavery Act Transparency Statement|Sitemap|About US| Contact US: help@patsnap.com