Hyperspectral Intelligent Classification Method Based on Prototype Learning Mechanism and Multidimensional Residual Network
A learning mechanism and hyperspectral technology, applied in computer parts, character and pattern recognition, instruments, etc., can solve the problems of deep model robustness, slow convergence speed, and low classification accuracy
- Summary
- Abstract
- Description
- Claims
- Application Information
AI Technical Summary
Problems solved by technology
Method used
Image
Examples
Embodiment Construction
[0060] Below in conjunction with the emulation experiment of specific embodiment and accompanying drawing, the present invention is described in further detail:
[0061] The hardware environment that the present invention implements simulation experiment is: Xeon(R)W-2123CPU@3.60GHz×8, memory 32GiB, GPU TITAN Xp; software platform: TensorFlow2.0 and keras 2.2.4.
[0062] The hyperspectral data set used in the simulation experiment of the present invention is the hyperspectral image of Pavel University. The dataset contains 103 bands with an image size of 610 × 340 pixels and a spatial resolution of 1.3m. The data set is marked with 9 types of ground objects according to the ground truth, and all categories are selected for training and testing in the simulation experiment.
[0063] refer to figure 1 , figure 2 and image 3 , to further describe in detail the specific steps of the present invention. Proceed as follows:
[0064] Step S1: Construct a multi-dimensional re...
PUM
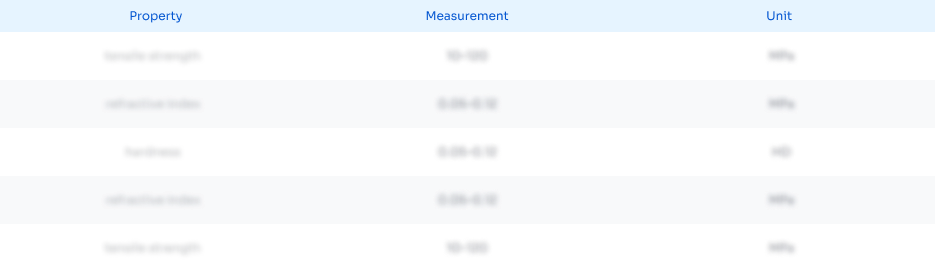
Abstract
Description
Claims
Application Information
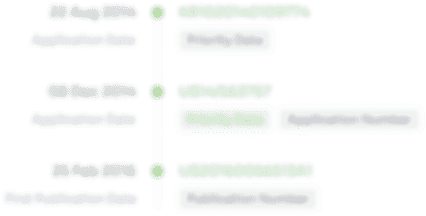
- R&D Engineer
- R&D Manager
- IP Professional
- Industry Leading Data Capabilities
- Powerful AI technology
- Patent DNA Extraction
Browse by: Latest US Patents, China's latest patents, Technical Efficacy Thesaurus, Application Domain, Technology Topic, Popular Technical Reports.
© 2024 PatSnap. All rights reserved.Legal|Privacy policy|Modern Slavery Act Transparency Statement|Sitemap|About US| Contact US: help@patsnap.com