Capsule network rapid routing method based on kernel density estimation and mean shift
A technology of kernel density estimation and mean shift, applied in the fields of deep learning and computer vision, it can solve problems such as blocking costs, and achieve the effect of improving time efficiency and recognition performance.
- Summary
- Abstract
- Description
- Claims
- Application Information
AI Technical Summary
Problems solved by technology
Method used
Image
Examples
Embodiment Construction
[0042] In order to make the object, technical solution and advantages of the present invention clearer, the present invention will be described in detail below in conjunction with the accompanying drawings and specific embodiments.
[0043] Such as figure 1 As shown, the present invention discloses a capsule network fast routing method based on kernel density estimation and mean shift, which mainly includes the following steps:
[0044] Step 1. According to the kernel density estimation formula, the probability density function for multi-clustering problems is given;
[0045] Step 2, based on the probability density function given in step 1, the dynamic routing process is modeled as an optimization problem;
[0046] Step 3, using the mean shift algorithm to solve the optimization problem in step 2;
[0047] In step 4, the result obtained in step 3 is used as the output of the capsule network to obtain the final classification result.
[0048] Steps 1-4 will be described in ...
PUM
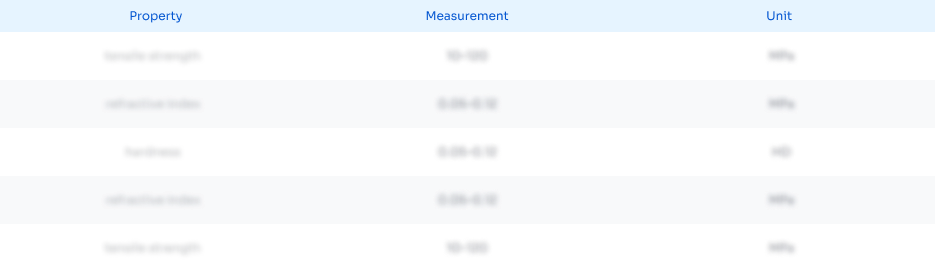
Abstract
Description
Claims
Application Information
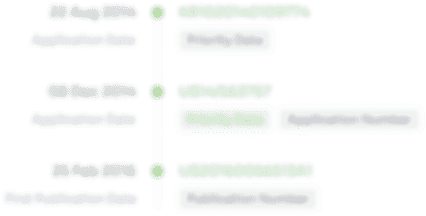
- R&D
- Intellectual Property
- Life Sciences
- Materials
- Tech Scout
- Unparalleled Data Quality
- Higher Quality Content
- 60% Fewer Hallucinations
Browse by: Latest US Patents, China's latest patents, Technical Efficacy Thesaurus, Application Domain, Technology Topic, Popular Technical Reports.
© 2025 PatSnap. All rights reserved.Legal|Privacy policy|Modern Slavery Act Transparency Statement|Sitemap|About US| Contact US: help@patsnap.com