Vehicle detection and identification method based on improved DSOD model
A vehicle detection and recognition method technology, applied in the field of vehicle detection and recognition of DSOD model, can solve the problems of slow calculation speed, low detection accuracy of network regression calculation, huge memory consumption, etc., and achieve the effect of reducing the amount of calculation
- Summary
- Abstract
- Description
- Claims
- Application Information
AI Technical Summary
Problems solved by technology
Method used
Image
Examples
Embodiment Construction
[0039] The technical solutions provided by the present invention will be further described below in conjunction with the accompanying drawings.
[0040] Embodiments of the present invention are described below through specific examples and related diagrams, and those skilled in the art can easily understand other advantages and effects of the present invention from the content disclosed in this specification. The present invention can also be verified or applied by means of other different types of implementations.
[0041] The embodiment of the present invention takes the KITTI data set jointly established by the Karlsruhe Institute of Technology in Germany and the Toyota American Institute of Technology as the research object. The KITTI dataset contains real image data collected from scenes such as urban areas, rural areas, and highways. There are up to 15 vehicles and 30 pedestrians in each image, as well as various degrees of occlusion and truncation.
[0042] see Figur...
PUM
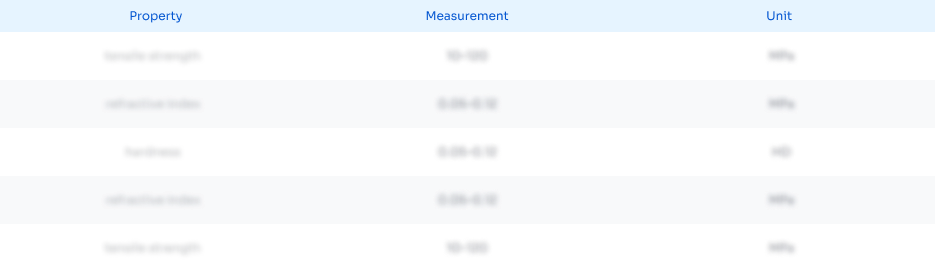
Abstract
Description
Claims
Application Information
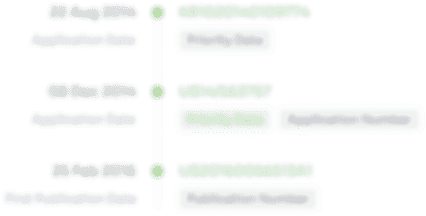
- R&D Engineer
- R&D Manager
- IP Professional
- Industry Leading Data Capabilities
- Powerful AI technology
- Patent DNA Extraction
Browse by: Latest US Patents, China's latest patents, Technical Efficacy Thesaurus, Application Domain, Technology Topic, Popular Technical Reports.
© 2024 PatSnap. All rights reserved.Legal|Privacy policy|Modern Slavery Act Transparency Statement|Sitemap|About US| Contact US: help@patsnap.com