Power load probabilistic prediction method based on chaotic population algorithm and Bayesian network
A Bayesian network and power load technology, applied in the field of power consumption, can solve the problems of not meeting the accuracy and availability requirements, not meeting the time availability requirements, and low prediction accuracy
- Summary
- Abstract
- Description
- Claims
- Application Information
AI Technical Summary
Problems solved by technology
Method used
Image
Examples
Embodiment Construction
[0080] In this embodiment, a probabilistic prediction method of power load based on chaotic crowd algorithm and Bayesian network, such as figure 1 As shown, including: obtaining the actual data of temperature, relative humidity, wind power and power load, and preprocessing the data; performing wavelet threshold de-drying processing on the original data of power load, restoring the real information of the time series of power load; establishing a Bayesian network model , to obtain the initial prediction interval; calculate the range of interval change amplitude, and use the chaotic crowd algorithm to obtain the optimal interval change range when the fitness function is optimal, so as to obtain the final prediction interval, and analyze and evaluate the prediction results. Specifically, proceed as follows:
[0081] Step 1. Obtain the actual values of air temperature, relative humidity, wind force and electric load and perform data preprocessing:
[0082] Step 1.1, collect the...
PUM
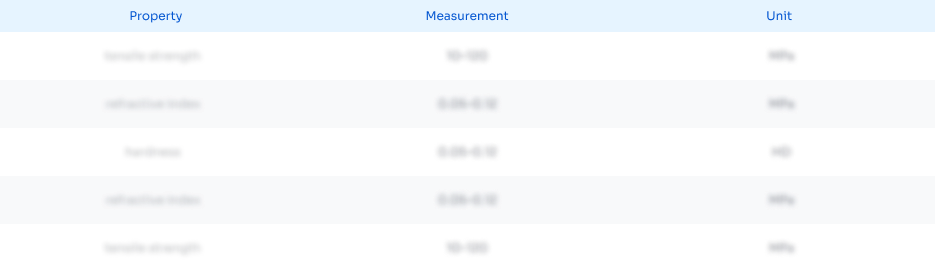
Abstract
Description
Claims
Application Information
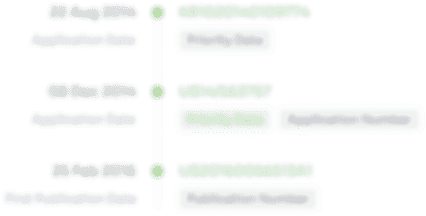
- R&D Engineer
- R&D Manager
- IP Professional
- Industry Leading Data Capabilities
- Powerful AI technology
- Patent DNA Extraction
Browse by: Latest US Patents, China's latest patents, Technical Efficacy Thesaurus, Application Domain, Technology Topic, Popular Technical Reports.
© 2024 PatSnap. All rights reserved.Legal|Privacy policy|Modern Slavery Act Transparency Statement|Sitemap|About US| Contact US: help@patsnap.com