Fine-grained image classification method based on sparse bilinear convolutional neural network
A technology of convolutional neural network and classification method, which is applied in the field of fine-grained image classification based on sparse bilinear convolutional neural network, can solve the problems of fitting, accuracy rate and accuracy rate, etc., to reduce parameters, Improve accuracy and prevent overfitting
- Summary
- Abstract
- Description
- Claims
- Application Information
AI Technical Summary
Problems solved by technology
Method used
Image
Examples
Embodiment Construction
[0029] A novel and simple pruning technique is used to cut the feature channel of the bilinear convolutional neural network. During the training process, the feature channel will be automatically sparse and the importance of the feature channel to the classification will be identified, and the size will be sorted according to the importance for proportional cutting. The pruning technology in the commonly used network model compression methods includes layer-level pruning, channel-level pruning, and weight-level pruning of the network model. Hierarchical cropping is too rough, it is not suitable for fine-grained image classification, and it is easy to lose important features. The calculation of weight-level cropping is too complicated, which will increase the complexity of the algorithm. Channel-level clipping strikes a balance between flexibility and ease of implementation. But common channel cropping techniques are not suitable for commonly used deep learning-based computer v...
PUM
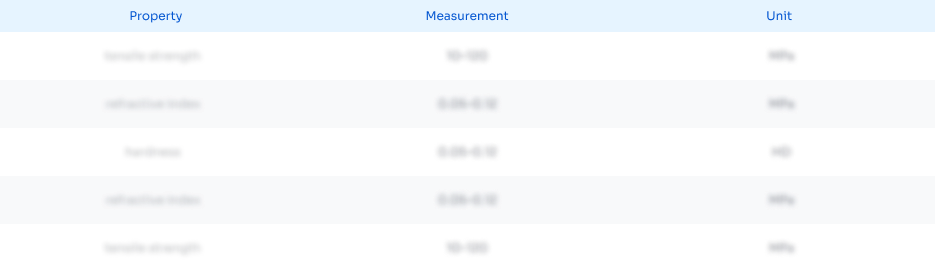
Abstract
Description
Claims
Application Information
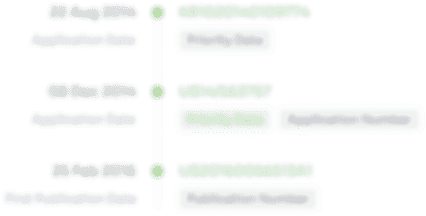
- R&D Engineer
- R&D Manager
- IP Professional
- Industry Leading Data Capabilities
- Powerful AI technology
- Patent DNA Extraction
Browse by: Latest US Patents, China's latest patents, Technical Efficacy Thesaurus, Application Domain, Technology Topic, Popular Technical Reports.
© 2024 PatSnap. All rights reserved.Legal|Privacy policy|Modern Slavery Act Transparency Statement|Sitemap|About US| Contact US: help@patsnap.com