Zero sample classification method based on dual-triple deep metric learning network
A technology of metric learning and classification methods, which is applied in the field of zero-sample classification based on the double-triple deep metric learning network, can solve the problem of not making full use of the differences and connections between visual features and semantic features of samples, and achieve simple structure implementation and training The effect of simple method and few training parameters
- Summary
- Abstract
- Description
- Claims
- Application Information
AI Technical Summary
Problems solved by technology
Method used
Image
Examples
Embodiment Construction
[0026] A zero-shot classification method based on a double-triple deep metric learning network of the present invention will be described in detail below with reference to the embodiments and drawings.
[0027] Such as figure 1 As shown, a zero-sample classification method based on a double-triple deep metric learning network of the present invention, first, utilizes a convolutional neural network to extract visual features of sample images; utilizes manually labeled attribute features as sample semantic features, and the method includes Training phase and testing phase: In the training phase, firstly, the semantic features of the training samples are input to the mapping network, and the output of the mapping network is sent to the visual space; in the visual space, a pair of training sample semantic features and training sample visual features belonging to the same category are selected to form Positive sample pair, and then select a training sample semantic feature of a dif...
PUM
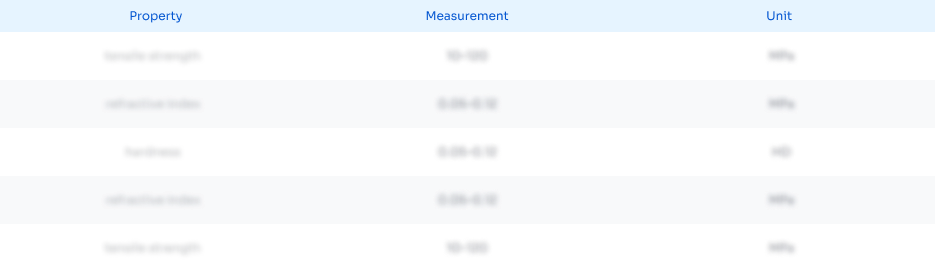
Abstract
Description
Claims
Application Information
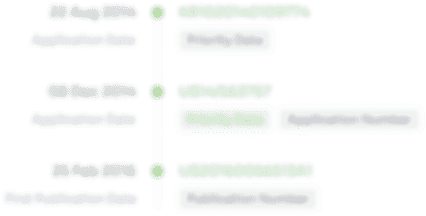
- R&D
- Intellectual Property
- Life Sciences
- Materials
- Tech Scout
- Unparalleled Data Quality
- Higher Quality Content
- 60% Fewer Hallucinations
Browse by: Latest US Patents, China's latest patents, Technical Efficacy Thesaurus, Application Domain, Technology Topic, Popular Technical Reports.
© 2025 PatSnap. All rights reserved.Legal|Privacy policy|Modern Slavery Act Transparency Statement|Sitemap|About US| Contact US: help@patsnap.com