A method and system for semi-supervised question-answer induction based on deep generative models
A technology for generating models and question-and-answer pairs, applied in the field of automatic question-answer pair induction methods and systems, can solve problems such as labor-intensive, low-information, and high-quality question-and-answer pairs are more difficult, and achieve the effect of improving the quality of training data
- Summary
- Abstract
- Description
- Claims
- Application Information
AI Technical Summary
Problems solved by technology
Method used
Image
Examples
Embodiment 1
[0093] figure 1 It is the overall flowchart of the semi-supervised question-answer induction method based on the deep generation model provided by the present invention, the method includes the following steps: S10, receiving dialogue data, and sorting out candidate questions and answers from the input dialogue data through the candidate question-answer pair generation method Right; S20, score the candidate question-answer pair by using the question-answer pair evaluation method based on the depth generation model; S30, obtain a high-quality question-answer pair by using the question-answer pair screening method according to the scoring result of the candidate question-answer pair; S40, the depth-based The generated model model is pre-trained in a semi-supervised learning manner and then applied to the question-answer pair evaluation method.
[0094] In the step S10 of candidate question-answer pair generation, the question-answer pair generation method will be used in the can...
Embodiment 2
[0141] Figure 6 is an example block diagram of the semi-supervised question-answer induction system based on the deep generation model of the present invention, the system includes:
[0142] Input module 10: for receiving dialogue data;
[0143] Candidate question-answer pair generation module 20: used to sort out candidate question-answer pair from the received dialogue data through the candidate question-answer pair generation model;
[0144] Question-answer pair evaluation module 30: used to score the candidate question-answer pair through a deep generation model, wherein the model is pre-trained by the training module;
[0145] Question-answer pair screening module 40: used to obtain high-quality question-answer pair through question-answer pair screening according to the scoring results of the candidate question-answer pair
[0146] Output module 50: used to provide high-quality question-answer pairs in the dialogue data according to the results of the question-answer ...
PUM
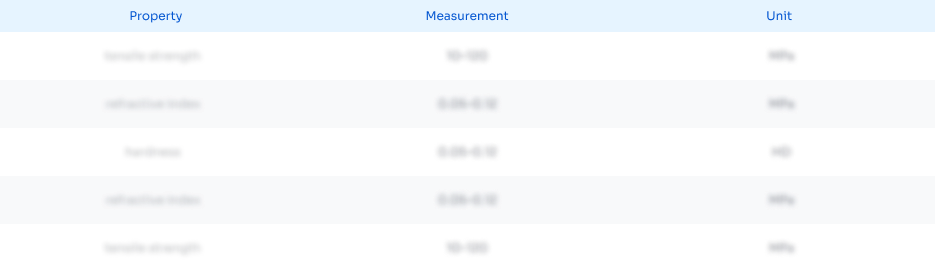
Abstract
Description
Claims
Application Information
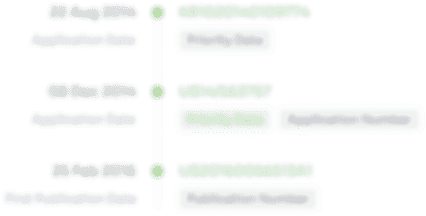
- R&D Engineer
- R&D Manager
- IP Professional
- Industry Leading Data Capabilities
- Powerful AI technology
- Patent DNA Extraction
Browse by: Latest US Patents, China's latest patents, Technical Efficacy Thesaurus, Application Domain, Technology Topic, Popular Technical Reports.
© 2024 PatSnap. All rights reserved.Legal|Privacy policy|Modern Slavery Act Transparency Statement|Sitemap|About US| Contact US: help@patsnap.com