Fault prediction method based on synthetic minority class oversampling and deep learning
A fault prediction and deep learning technology, applied in neural learning methods, character and pattern recognition, instruments, etc., can solve problems such as the inability to overcome the problem of unbalanced data set data distribution
- Summary
- Abstract
- Description
- Claims
- Application Information
AI Technical Summary
Problems solved by technology
Method used
Image
Examples
Embodiment 1
[0042] In order to solve the problems in the existing technology, such as the lack of a solution to the inability to carry out deep learning predictive analysis in the case of few fault samples, the blindness of neighbor selection in the traditional SMOTE method, and the reduction of the marginalization of the distribution of unbalanced data sets, the electromechanical equipment is in operation. When an abnormality occurs, it is impossible to effectively predict the problematic equipment or components. This embodiment provides a fault prediction method based on synthetic minority class oversampling and deep learning. see figure 1 , which is a flowchart of a K-Means-SMOTE improved resampling method for unbalanced data classification of electromechanical equipment provided in this embodiment. This method first uses the K-Means method to cluster the minority class samples in the sample set, and deletes the noise sample class whose centroid of each cluster is closest to the majori...
Embodiment 2
[0085] In the following, the above process will be described in detail in combination with specific examples.
[0086] Specifically, taking a certain electromechanical equipment failure ("pump speed output failure prediction") as an example, the failure prediction based on deep learning is carried out.
[0087] 1) Preprocess the original data of the fault, clean the data to remove invalid data and duplicate data, import the processed data, and divide them into majority class samples and minority class samples, and establish parameters in normal state samples; use the K-Means method Clustering minority class samples;
[0088] 2) Find out the noise clusters, and remove new noise clusters in the minority class sample set P;
[0089] 3) Reclassify each remaining cluster of the minority class samples, and delete the noise class samples in each remaining class cluster of the minority class;
[0090] 4) Synthesize new samples and merge data, the merged data is as follows image 3 ...
PUM
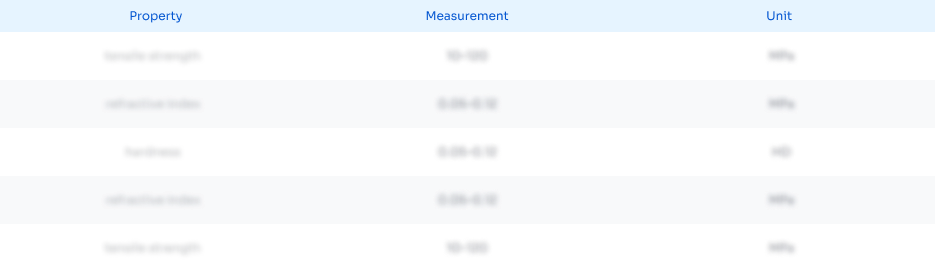
Abstract
Description
Claims
Application Information
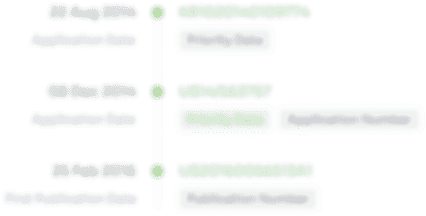
- R&D Engineer
- R&D Manager
- IP Professional
- Industry Leading Data Capabilities
- Powerful AI technology
- Patent DNA Extraction
Browse by: Latest US Patents, China's latest patents, Technical Efficacy Thesaurus, Application Domain, Technology Topic, Popular Technical Reports.
© 2024 PatSnap. All rights reserved.Legal|Privacy policy|Modern Slavery Act Transparency Statement|Sitemap|About US| Contact US: help@patsnap.com