An asphalt road crack image segmentation method based on a generative adversarial network
A technology of image segmentation and highway, applied in image analysis, biological neural network model, image data processing, etc., can solve the problems of large amount of labeled data, low recall rate, high model accuracy rate, etc., and achieve good generalization ability and robustness Effect
- Summary
- Abstract
- Description
- Claims
- Application Information
AI Technical Summary
Problems solved by technology
Method used
Image
Examples
specific Embodiment
[0033] The implementation steps are as follows:
[0034] Step 1. Dataset preprocessing
[0035] Step 1.1: Build a model dataset. The size of the collected asphalt road crack image is 3040 pixels × 2048 pixels, and 100 images are randomly selected to form the original data set; the crack area is manually marked on the original data set, and the crack area is marked in black according to the actual size of the crack in the image. , the rest of the background area is marked in white, and the target data set is formed based on the relabeled data of the original data set.
[0036] Step 1.2: Image data generation. Due to the small amount of data in the original dataset in step 1.1, image data augmentation is required before training the model. Before training the model, the same random rotation transformation, random flip transformation, and random translation transformation are performed on the original data set and the target data set in step 1.1 to obtain the model training da...
PUM
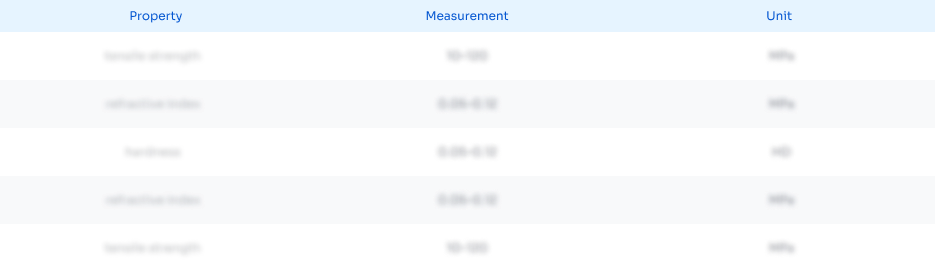
Abstract
Description
Claims
Application Information
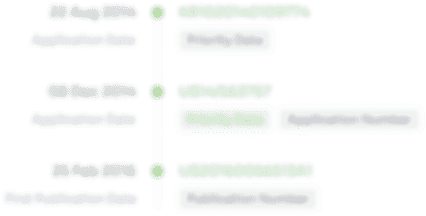
- R&D Engineer
- R&D Manager
- IP Professional
- Industry Leading Data Capabilities
- Powerful AI technology
- Patent DNA Extraction
Browse by: Latest US Patents, China's latest patents, Technical Efficacy Thesaurus, Application Domain, Technology Topic, Popular Technical Reports.
© 2024 PatSnap. All rights reserved.Legal|Privacy policy|Modern Slavery Act Transparency Statement|Sitemap|About US| Contact US: help@patsnap.com