A multi-class EEG classification method based on dual-rule active extreme learning machine
An ultra-limited learning machine and classification method technology, which is applied in the field of multi-class motor imagery task classification and motor imagery EEG signal pattern recognition. high accuracy effect
- Summary
- Abstract
- Description
- Claims
- Application Information
AI Technical Summary
Problems solved by technology
Method used
Image
Examples
Embodiment Construction
[0036] The motor imagery EEG classification method based on the dual-rule active extreme learning machine of the present invention will be described in detail below in conjunction with the accompanying drawings.
[0037] Each step will be described in detail below one by one.
[0038] Step 1: Extract features from the EEG signal, such as figure 1 shown;
[0039] Specifically: use the one-to-many common space mode (OVR-CSP) algorithm to extract the features of the original multi-type EEG signals, and obtain new EEG feature samples {X, Y}={{X l ,Y l},X u}, where X is all training samples; x l for n l labeled training samples; Y l for n l Labels corresponding to labeled training samples; x u for n u unlabeled training samples.
[0040] Step 2: Train the initial extreme learning machine (ELM) classifier, such as figure 2 shown;
[0041] Specifically: According to a small number of labeled training samples {X l ,Y l}, calculate the initial output weight β 0 , to...
PUM
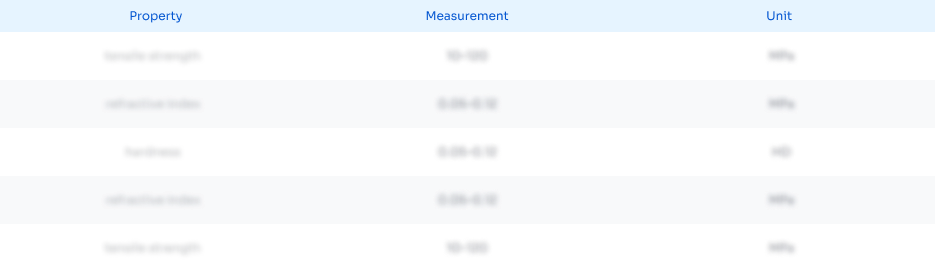
Abstract
Description
Claims
Application Information
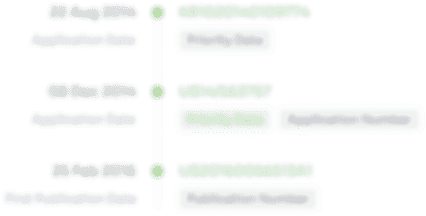
- R&D Engineer
- R&D Manager
- IP Professional
- Industry Leading Data Capabilities
- Powerful AI technology
- Patent DNA Extraction
Browse by: Latest US Patents, China's latest patents, Technical Efficacy Thesaurus, Application Domain, Technology Topic, Popular Technical Reports.
© 2024 PatSnap. All rights reserved.Legal|Privacy policy|Modern Slavery Act Transparency Statement|Sitemap|About US| Contact US: help@patsnap.com