Classification method based on correlation entropy and transfer learning
A technology of transfer learning and classification methods, applied in the field of machine learning, can solve the problems of insufficient labeled samples in the target data set, and achieve the effects of solving insufficient labeled samples, solving the lack of convergence, and enhancing robustness
- Summary
- Abstract
- Description
- Claims
- Application Information
AI Technical Summary
Problems solved by technology
Method used
Image
Examples
Embodiment Construction
[0039] In order to make the above-mentioned features and advantages of the present invention more comprehensible, the following specific embodiments are described in detail in conjunction with the accompanying drawings.
[0040] This embodiment provides a classification method based on correlation entropy and migration learning, such as figure 1 The flow chart shown, the steps include:
[0041] S1: Preprocess and normalize the labeled source data set and unlabeled target data set.
[0042] In this embodiment, the standard CMU-PIE face database is used as the experimental data. The CMU-PIE face database contains 41368 face images from 68 individuals. These images come from different angles of the face, and the size of each image is 32*32. In this embodiment, the labeled source data set comes from the image in CMU-PIE whose face angle is the left face, and the unlabeled target data set comes from the image in CMU-PIE whose face angle is the right face. figure 2 is a schemat...
PUM
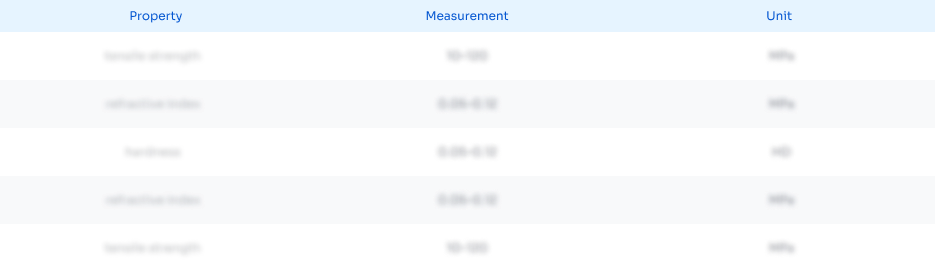
Abstract
Description
Claims
Application Information
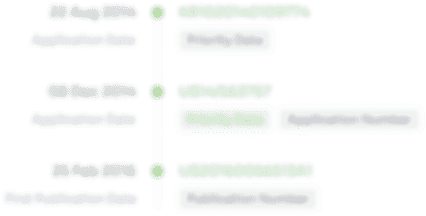
- Generate Ideas
- Intellectual Property
- Life Sciences
- Materials
- Tech Scout
- Unparalleled Data Quality
- Higher Quality Content
- 60% Fewer Hallucinations
Browse by: Latest US Patents, China's latest patents, Technical Efficacy Thesaurus, Application Domain, Technology Topic, Popular Technical Reports.
© 2025 PatSnap. All rights reserved.Legal|Privacy policy|Modern Slavery Act Transparency Statement|Sitemap|About US| Contact US: help@patsnap.com