Medical image synthesis method based on generation countermeasure network
A synthesis method and medical image technology, applied in the field of medical image synthesis based on generative confrontation network, can solve problems such as low quality of generation, unreasonable image synthesis, and low resolution of healthy images
- Summary
- Abstract
- Description
- Claims
- Application Information
AI Technical Summary
Problems solved by technology
Method used
Image
Examples
Embodiment Construction
[0042] In order to make the purpose, technical solutions and advantages of the present invention clearer, the implementation modes of the present invention will be further described in detail below in conjunction with the accompanying drawings:
[0043] according to figure 1 , the implementation method of medical image synthesis mainly has the following four steps:
[0044] Step 1: According to figure 1 , to obtain the real lesion image x a Corresponding health image G A2N (x a ).
[0045] Real lesion image x a via generator G A2N , after 3 convolution operations, 9 residual block convolution operations, then 2 deconvolution operations, and finally a convolution operation, adding to the original lesion image to obtain the real lesion image size of the input A consistent healthy image, the resulting healthy image is determined by the following expression:
[0046]
[0047] In order to ensure the invariance of non-lesional regions, a fidelity term loss function is add...
PUM
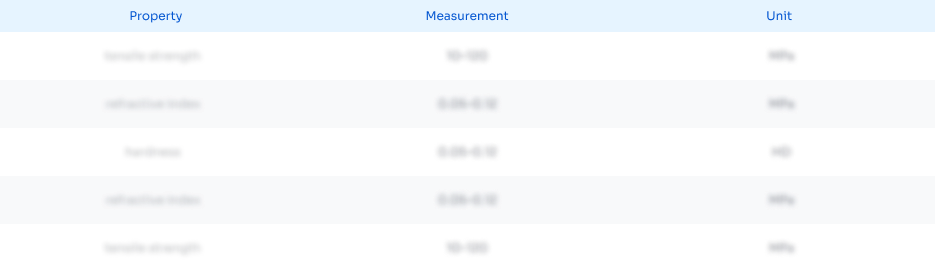
Abstract
Description
Claims
Application Information
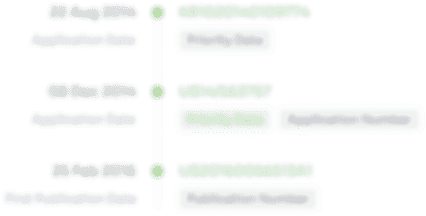
- R&D Engineer
- R&D Manager
- IP Professional
- Industry Leading Data Capabilities
- Powerful AI technology
- Patent DNA Extraction
Browse by: Latest US Patents, China's latest patents, Technical Efficacy Thesaurus, Application Domain, Technology Topic, Popular Technical Reports.
© 2024 PatSnap. All rights reserved.Legal|Privacy policy|Modern Slavery Act Transparency Statement|Sitemap|About US| Contact US: help@patsnap.com