DCNN (Deep Convolutional Neural Network) based radar radiation source identification method
A technology of deep convolution and neural network, applied in the field of radar radiation source identification based on deep convolutional neural network, can solve the problems of increasing the difficulty of neural network in radar recognition algorithm, generalization ability constraints, etc.
- Summary
- Abstract
- Description
- Claims
- Application Information
AI Technical Summary
Problems solved by technology
Method used
Image
Examples
specific Embodiment approach
[0071] The present invention proposes a radar radiation source identification method based on a deep convolutional neural network. According to the flow of the method below, and in conjunction with the accompanying drawings, the specific implementation is as follows:
[0072] 1. Data generation:
[0073] Such as figure 1 , the present invention selects 8 kinds of radar signals, which are linear frequency modulation signal (LFM), linear frequency modulation continuous wave (LFMCW), LFM-BC signal, Frank-LFM signal, S-type NLFM signal, Costas coded signal, P3 code coded signal, FSK / PSK signal. As described in step (1), change the value of the bandwidth B for the five types of signals LFM, LFMCW, LFM-BC, Frank-LFM, and S-type NLFM, thereby changing the size of the frequency modulation slope μ=B / T, and generating five types of data samples; the Costas codes and SK / PSK codes are fully arranged to generate two types of data samples; for P3 coded signals, ensure that Ncτc=25 to gene...
PUM
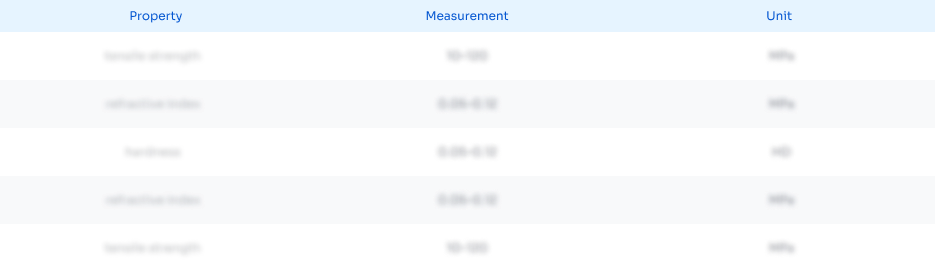
Abstract
Description
Claims
Application Information
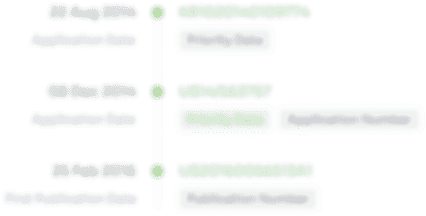
- Generate Ideas
- Intellectual Property
- Life Sciences
- Materials
- Tech Scout
- Unparalleled Data Quality
- Higher Quality Content
- 60% Fewer Hallucinations
Browse by: Latest US Patents, China's latest patents, Technical Efficacy Thesaurus, Application Domain, Technology Topic, Popular Technical Reports.
© 2025 PatSnap. All rights reserved.Legal|Privacy policy|Modern Slavery Act Transparency Statement|Sitemap|About US| Contact US: help@patsnap.com