Deep learning wind power alarm information analysis method based on small sample data
A deep learning and alarm information technology, applied in data processing applications, instruments, biological neural network models, etc., can solve problems such as far away, different data types, complex interrelationships, etc., to achieve improved accuracy, good expansion performance, The effect of simple online learning process
- Summary
- Abstract
- Description
- Claims
- Application Information
AI Technical Summary
Problems solved by technology
Method used
Image
Examples
Embodiment Construction
[0043] The technical solutions of the present invention will be further specifically described below through the embodiments and in conjunction with the accompanying drawings.
[0044] Examples of the described embodiments are shown in the drawings, wherein like or similar reference numerals designate like or similar elements or elements having the same or similar functions throughout. The embodiments described below by referring to the figures are exemplary only for explaining the present invention and should not be construed as limiting the present invention.
[0045] The following disclosure provides many different embodiments or examples for implementing different structures of the present invention. To simplify the disclosure of the present invention, components and arrangements of specific examples are described below. They are examples only and are not intended to limit the invention. Furthermore, the present invention may repeat reference numerals and / or letters in d...
PUM
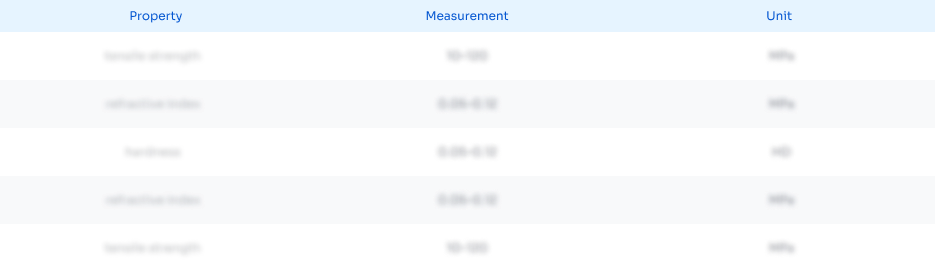
Abstract
Description
Claims
Application Information
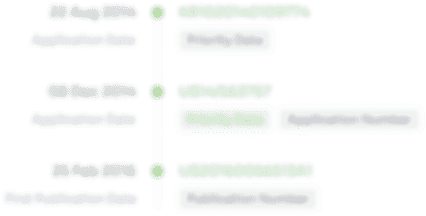
- R&D Engineer
- R&D Manager
- IP Professional
- Industry Leading Data Capabilities
- Powerful AI technology
- Patent DNA Extraction
Browse by: Latest US Patents, China's latest patents, Technical Efficacy Thesaurus, Application Domain, Technology Topic, Popular Technical Reports.
© 2024 PatSnap. All rights reserved.Legal|Privacy policy|Modern Slavery Act Transparency Statement|Sitemap|About US| Contact US: help@patsnap.com