A zero sample learning method based on a self-coding generative adversarial network
A sample learning and self-encoding technology, applied in neural learning methods, biological neural network models, computer components, etc., can solve problems such as weakening the alignment relationship of different modalities and ignoring them
- Summary
- Abstract
- Description
- Claims
- Application Information
AI Technical Summary
Problems solved by technology
Method used
Image
Examples
Embodiment Construction
[0032] The zero-shot learning method based on the self-encoder confrontation generative network of the present invention will be described in detail below with reference to the embodiments and the accompanying drawings.
[0033] The zero-shot learning method based on the self-encoding confrontation generation network of the present invention uses the visual sample data of visible categories and the corresponding category semantic features to train a kind of confrontation generation network based on the self-encoder framework, and its structure diagram is as follows figure 1 shown. The method includes the following steps:
[0034] 1) Input the visual feature x of the visible category sample into the encoder, and obtain the hidden category semantic feature under the supervision of the category semantic feature a corresponding to the sample and latent noise features The encoder is composed of a three-layer network, and the encoder structure is: fully connected layer-hidden la...
PUM
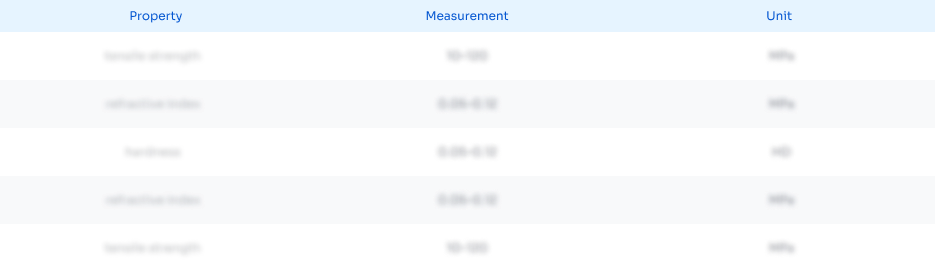
Abstract
Description
Claims
Application Information
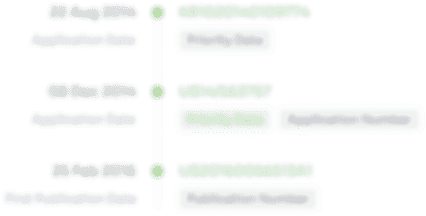
- R&D Engineer
- R&D Manager
- IP Professional
- Industry Leading Data Capabilities
- Powerful AI technology
- Patent DNA Extraction
Browse by: Latest US Patents, China's latest patents, Technical Efficacy Thesaurus, Application Domain, Technology Topic, Popular Technical Reports.
© 2024 PatSnap. All rights reserved.Legal|Privacy policy|Modern Slavery Act Transparency Statement|Sitemap|About US| Contact US: help@patsnap.com