A cluster network super-resolution image reconstruction method of an Laplace pyramid structure
A pyramid structure, low-resolution image technology, applied in image data processing, image image conversion, neural learning methods, etc., can solve the problems of ignoring the feedback and communication information of the convolutional layer, increasing computing costs, and weakening visible artifacts. Achieve the effect of solving super-resolution image reconstruction, avoiding gradient explosion, and dense inter-layer connections
- Summary
- Abstract
- Description
- Claims
- Application Information
AI Technical Summary
Problems solved by technology
Method used
Image
Examples
Embodiment Construction
[0054] The present invention will be described in further detail below in conjunction with examples and accompanying drawings, but the protection scope of the present invention is not limited thereto.
[0055] The present invention relates to a clique network super-resolution image reconstruction method of a Laplacian pyramid structure. There are forward and feedback connections between any two convolutional layers in the same building block CNB in the present invention. The information is updated alternately so that the information flow and feedback mechanism can be maximized, and the connections between layers are denser. At the same time, the Laplacian pyramid structure is used to gradually optimize the reconstruction results by gradually reconstructing high-resolution images, and the residual Learning is applied to the network, reducing network parameters and avoiding gradient explosion.
[0056] figure 1 It is a flowchart for the implementation of the present invention...
PUM
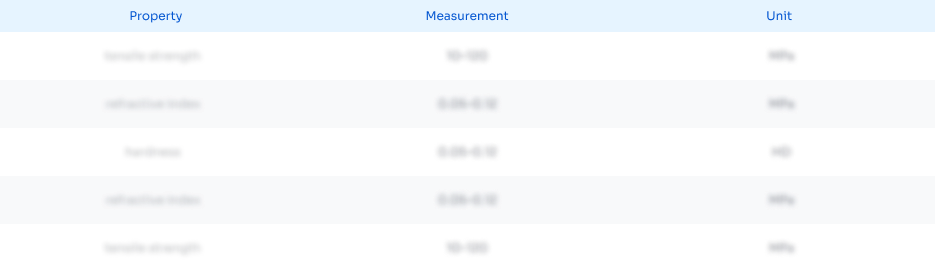
Abstract
Description
Claims
Application Information
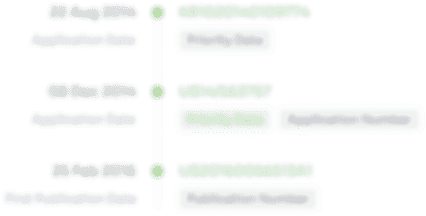
- R&D Engineer
- R&D Manager
- IP Professional
- Industry Leading Data Capabilities
- Powerful AI technology
- Patent DNA Extraction
Browse by: Latest US Patents, China's latest patents, Technical Efficacy Thesaurus, Application Domain, Technology Topic, Popular Technical Reports.
© 2024 PatSnap. All rights reserved.Legal|Privacy policy|Modern Slavery Act Transparency Statement|Sitemap|About US| Contact US: help@patsnap.com