Pedestrian flow statistics method based on deep learning and multi-target tracking
A multi-target tracking and deep learning technology, applied in neural learning methods, computing, computer components, etc., can solve the problems of low pedestrian flow statistics accuracy, error-prone, disordered tracking, etc., and achieve accurate pedestrian flow statistics. Easy to track chaotic, tracked trajectories with accurate effects
- Summary
- Abstract
- Description
- Claims
- Application Information
AI Technical Summary
Problems solved by technology
Method used
Image
Examples
Embodiment
[0049] A method for counting pedestrian flow based on deep learning and multi-target tracking, comprising the following steps:
[0050] S1: Shoot pedestrian monitoring video in real time, and read images in continuous video frames of pedestrian monitoring video. It should be noted that in this step, it is possible, but not limited to, to use a network surveillance camera to shoot pedestrian surveillance videos. Just take the video image.
[0051] S2: Set the effective area of the image in the continuous video frame, and set the flow count whose initial value is 0. It should be noted that the setting of the effective area is based on the observation requirements, and the non-interest areas that are likely to affect the pedestrian statistics are filled with black to achieve the purpose of removing the noise generated by the surrounding environment.
[0052] S3: Construct a pedestrian detection model based on deep learning and train it; preferably, in the step S3, the pedestr...
PUM
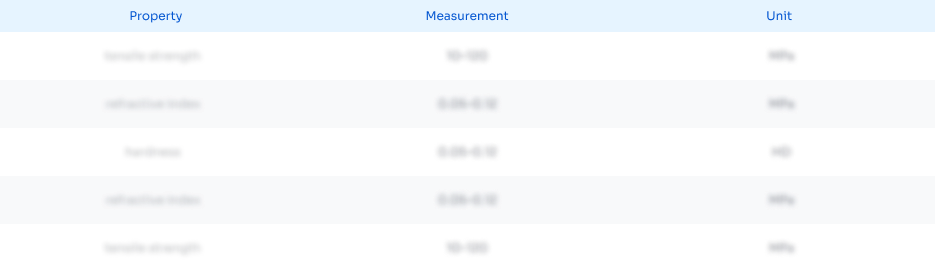
Abstract
Description
Claims
Application Information
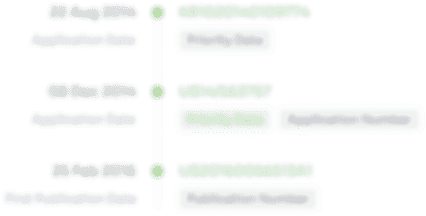
- R&D Engineer
- R&D Manager
- IP Professional
- Industry Leading Data Capabilities
- Powerful AI technology
- Patent DNA Extraction
Browse by: Latest US Patents, China's latest patents, Technical Efficacy Thesaurus, Application Domain, Technology Topic, Popular Technical Reports.
© 2024 PatSnap. All rights reserved.Legal|Privacy policy|Modern Slavery Act Transparency Statement|Sitemap|About US| Contact US: help@patsnap.com