An aircraft path prediction method based on depth memory network
A track prediction and aircraft technology, applied in the field of civil aviation, can solve problems such as low learning efficiency, poor prediction accuracy, and affecting prediction quality
- Summary
- Abstract
- Description
- Claims
- Application Information
AI Technical Summary
Problems solved by technology
Method used
Image
Examples
Embodiment Construction
[0068] The technical solutions of the present invention will be further described below in conjunction with the accompanying drawings and embodiments.
[0069] A kind of aircraft trajectory prediction method based on deep memory network of the present invention, this method comprises the steps:
[0070] (1) Extracting the data set of aircraft track information and performing data cleaning, and performing data specification on the cleaned aircraft track information data set to form a new data set of aircraft track information;
[0071] (2) according to the new aircraft track information data set that step (1) forms, construct input and output sample vector, carry out standardization process to input and output sample vector, generate dimensionless training data set;
[0072] (3) according to the dimensionless training data set that step (2) generates, construct deep memory network model;
[0073] (4) Use the deep network prediction model built in step (3) to predict the aircra...
PUM
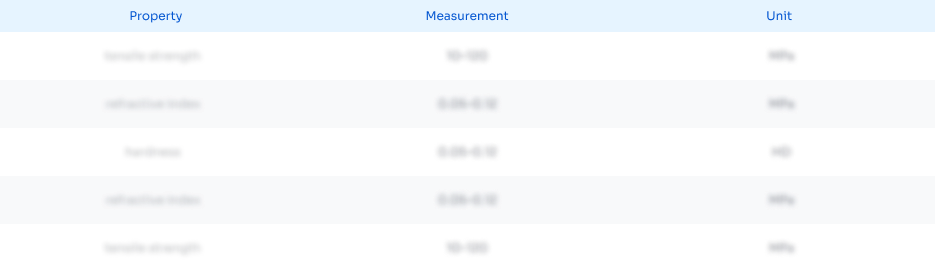
Abstract
Description
Claims
Application Information
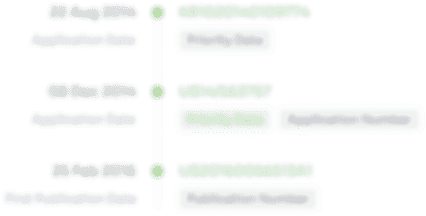
- R&D Engineer
- R&D Manager
- IP Professional
- Industry Leading Data Capabilities
- Powerful AI technology
- Patent DNA Extraction
Browse by: Latest US Patents, China's latest patents, Technical Efficacy Thesaurus, Application Domain, Technology Topic, Popular Technical Reports.
© 2024 PatSnap. All rights reserved.Legal|Privacy policy|Modern Slavery Act Transparency Statement|Sitemap|About US| Contact US: help@patsnap.com