Network traffic resource situation predication method based on long short-term memory LSTM model
A prediction method and technology of network traffic, applied in the direction of data exchange network, digital transmission system, electrical components, etc., can solve the problems of difficult prediction error, strong randomness of data, and difficulty in finding modeling methods, so as to improve prediction accuracy, Significant effect
- Summary
- Abstract
- Description
- Claims
- Application Information
AI Technical Summary
Problems solved by technology
Method used
Image
Examples
Embodiment
[0054] In order to test the effect of the traffic prediction model, this example uses Abilene network traffic data for testing. The Abilene network is an American education and research network. Its core network topology includes 12 nodes and 15 bidirectional links. The traffic flow transmitted between source and destination nodes (OD pairs) is sampled every 5 minutes. The experiment selected real network traffic data from 2003 / 05 / 01 to 2003 / 05 / 30, with 12*24*7*4=8064 traffic matrices in total. .
[0055] S1. First, the traffic data is preprocessed. From the collected 8064 flow matrices, the flow values at all moments between any two nodes are extracted to form a time series, and part of the time series is selected for prediction.
[0056] Since the time granularity may affect the prediction results, the extracted time series are further sampled and aggregated to obtain a coarser-grained (and generally more stable) real flow value. figure 1 Take OD123 as an example for th...
PUM
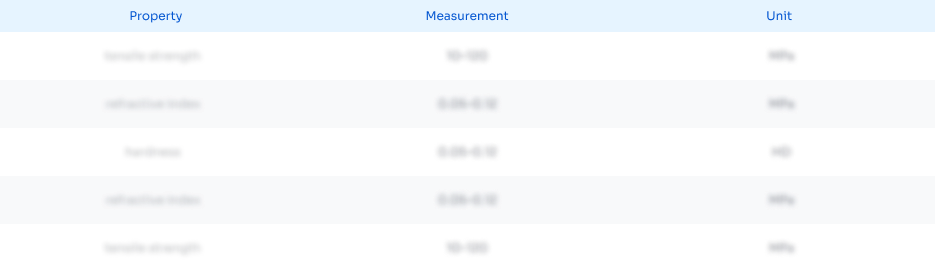
Abstract
Description
Claims
Application Information
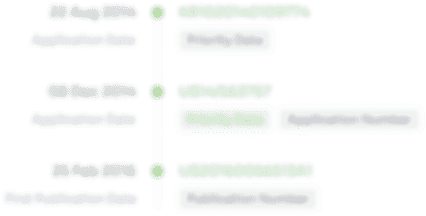
- R&D Engineer
- R&D Manager
- IP Professional
- Industry Leading Data Capabilities
- Powerful AI technology
- Patent DNA Extraction
Browse by: Latest US Patents, China's latest patents, Technical Efficacy Thesaurus, Application Domain, Technology Topic, Popular Technical Reports.
© 2024 PatSnap. All rights reserved.Legal|Privacy policy|Modern Slavery Act Transparency Statement|Sitemap|About US| Contact US: help@patsnap.com