Feature extraction method, device and server, and computer readable memory medium
A feature extraction and feature set technology, applied in the field of mobile Internet, can solve the problems of consumption, large number of feature bases, large memory resources, etc.
- Summary
- Abstract
- Description
- Claims
- Application Information
AI Technical Summary
Problems solved by technology
Method used
Image
Examples
Embodiment 1
[0076] Such as figure 2 As shown, an embodiment of the present invention provides a feature extraction method, which includes:
[0077] S201: Perform feature pre-grouping on the pre-training data set on the wireless side and the user side according to feature relevance to obtain initialization model parameters.
[0078] Specifically, the feature extraction server selects a part of the wireless side data and user side data of the mobile network as the pre-training data set. The selected pre-training data set is preprocessed to initially form the feature support-subsidiary feature set of the data and the corresponding support feature set and the corresponding feature shrinkage variable set.
[0079] This step S201 can be implemented as follows: input the pre-training data set into the feature selector to perform feature correlation calculation; according to the feature correlation calculation result, each data feature of the pre-training data set is classified into the corresponding s...
Embodiment 2
[0087] Such as image 3 As shown, a pre-training data set pre-grouping method provided by an embodiment of the present invention includes:
[0088] S2011: Input the pre-training data set into the feature selector to perform feature correlation calculation.
[0089] Among them, suppose the number of initial groups γ of the feature selector, the maximum number of groups γ max , The associated parameter set {κ A , Κ B , Κ C }, γ and number γ max Is a natural number, and γ max , Κ A Represents the irrelevant threshold, κ B Represents the weak correlation threshold, κ C Represents the strong correlation threshold, and 0≤κ A B C ≤1.
[0090] Assuming that κ is set in advance A =0.1, κ B = 0.3, κ C =0.9, and the number of groups γ=10 and the maximum number of groups γ max =15, select a part of data from the wireless side data and user side data of the mobile network as the pre-training data set, and input the pre-training data set into the feature selector.
[0091] When the feature va...
Embodiment 3
[0112] Such as Figure 4 As shown, a training data set grouping method provided by an embodiment of the present invention includes:
[0113] S2021: Input the training data set and the initialization model parameters into the feature selector, and perform group feature correlation calculation on each data feature of the training data set.
[0114] Specifically, the newly-added wireless side and user side training data sets and initialization model parameters are input to the feature selector.
[0115] When the grouped feature value is linear data, the following formula is used to calculate the group feature association for each data feature of the training data set:
[0116]
[0117] Where f j Represents the data feature, j represents the data feature number in the training data set; Represents the label of the grouping feature, i represents the number of the group; Representation feature f j Group with Characteristic correlation coefficient of Representation feature f j Group with ...
PUM
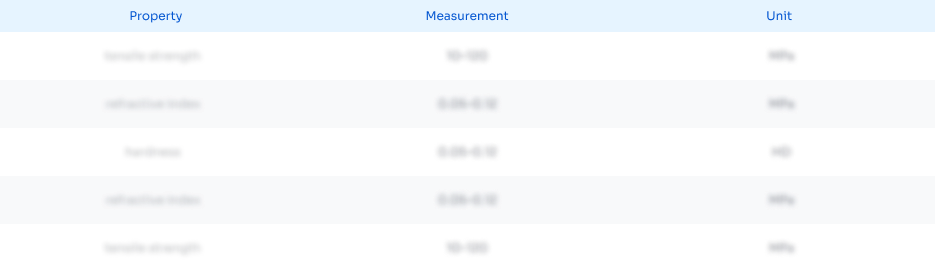
Abstract
Description
Claims
Application Information
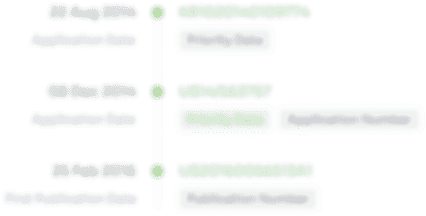
- R&D Engineer
- R&D Manager
- IP Professional
- Industry Leading Data Capabilities
- Powerful AI technology
- Patent DNA Extraction
Browse by: Latest US Patents, China's latest patents, Technical Efficacy Thesaurus, Application Domain, Technology Topic, Popular Technical Reports.
© 2024 PatSnap. All rights reserved.Legal|Privacy policy|Modern Slavery Act Transparency Statement|Sitemap|About US| Contact US: help@patsnap.com