Training method, identification method, device and processing equipment of recurrent neural network
A technology of recurrent neural network and training method, applied in the field of recognition method, device and processing equipment, and training method of recurrent neural network, can solve problems such as inability to learn action sequence conversion relationship, inability to recognize and predict action, and no effective solution is proposed.
- Summary
- Abstract
- Description
- Claims
- Application Information
AI Technical Summary
Problems solved by technology
Method used
Image
Examples
Embodiment 1
[0033] First, refer to figure 1 An example electronic system 100 for implementing the action recognition method, device, processing device and storage medium of the embodiment of the present invention will be described.
[0034] Such asfigure 1 A schematic structural diagram of an electronic system is shown, and the electronic system 100 includes one or more processing devices 102 and one or more storage devices 104 . Optionally, figure 1 The illustrated electronic system 100 may also include an input device 106, an output device 108, and a data acquisition device 110, and these components are interconnected by a bus system 112 and / or other forms of connection mechanisms (not shown). It should be noted that figure 1 The components and structures of the electronic system 100 shown are exemplary rather than limiting, and the electronic system may also have other components and structures as required.
[0035] The processing device 102 may be a gateway, or an intelligent termi...
Embodiment 2
[0043] According to an embodiment of the present invention, an embodiment of an action recognition method is provided. It should be noted that the steps shown in the flowcharts of the accompanying drawings can be executed in a computer system such as a set of computer-executable instructions, and, although A logical order is shown in the flowcharts, but in some cases the steps shown or described may be performed in an order different from that shown or described herein.
[0044] The recursive neural network used in the embodiment of the present invention is trained based on the action recognition network training method of the CTC (dynamic programming method connection time series classification method, Connectionist temporal classification) loss function, see figure 2 The flowchart of the shown recursive neural network training method, the method specifically includes the following steps:
[0045] Step S202, acquiring training samples. The training samples include multi-fra...
Embodiment 3
[0069] Corresponding to the training method of the recurrent neural network provided in the second embodiment, the embodiment of the present invention provides a training device of the recurrent neural network, see Figure 5 A structural block diagram of a training device for a recursive neural network shown, including:
[0070] The sample acquisition module 502 is used to acquire a training sample, the training sample includes a multi-frame image sequence of the video and an action identification corresponding to the video;
[0071] Feature extraction module 504, is used for carrying out feature extraction to multi-frame image sequence, obtains image sequence feature, and image sequence feature comprises the feature of each frame of image;
[0072] The action classification module 506 is used to input the image sequence feature into the recurrent neural network to carry out action classification, and obtain the action classification probability of each frame of image; wherein...
PUM
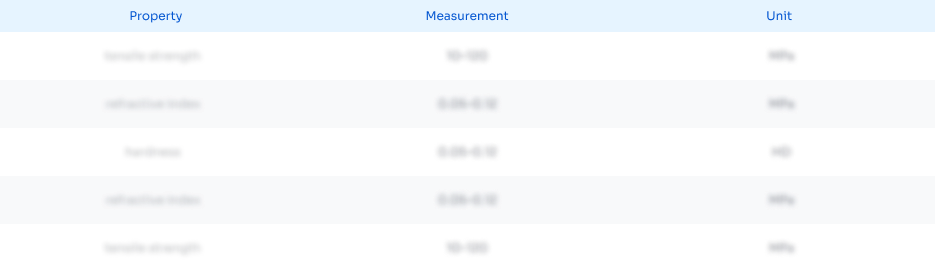
Abstract
Description
Claims
Application Information
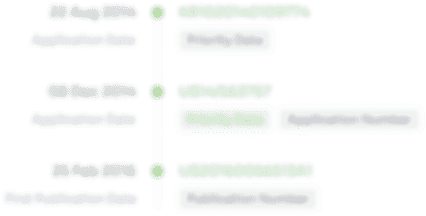
- R&D Engineer
- R&D Manager
- IP Professional
- Industry Leading Data Capabilities
- Powerful AI technology
- Patent DNA Extraction
Browse by: Latest US Patents, China's latest patents, Technical Efficacy Thesaurus, Application Domain, Technology Topic, Popular Technical Reports.
© 2024 PatSnap. All rights reserved.Legal|Privacy policy|Modern Slavery Act Transparency Statement|Sitemap|About US| Contact US: help@patsnap.com