Hyperspectral image classification method based on extended morphology and active learning
A technology of active learning and classification methods, applied in the field of hyperspectral classification based on extended morphology and active learning, can solve problems such as time-consuming, complicated calculation process, and inability to fully mine the spatial information of hyperspectral images, achieving short-term and improved The effect of classification accuracy
- Summary
- Abstract
- Description
- Claims
- Application Information
AI Technical Summary
Problems solved by technology
Method used
Image
Examples
Embodiment Construction
[0026] The present invention will be further described below in conjunction with the accompanying drawings.
[0027] Refer to attached figure 1 , the concrete steps of the present invention are as follows:
[0028] Step 1, input data;
[0029] Input a hyperspectral image to be classified and its corresponding image data set, which contains the spectral information and category labels of the data samples;
[0030] In the embodiment of the present invention, two hyperspectral images are selected to conduct two experiments. The first image is the Pavia_U hyperspectral image with 103 bands and the category label of the image; the second image is the IndianaPines hyperspectral image with 200 bands and the category label of the image;
[0031] Step 2, extracting spectral features;
[0032] Since the high-dimensional characteristics of hyperspectral images will bring problems such as complex calculations and redundant information, the present invention adopts the principal compon...
PUM
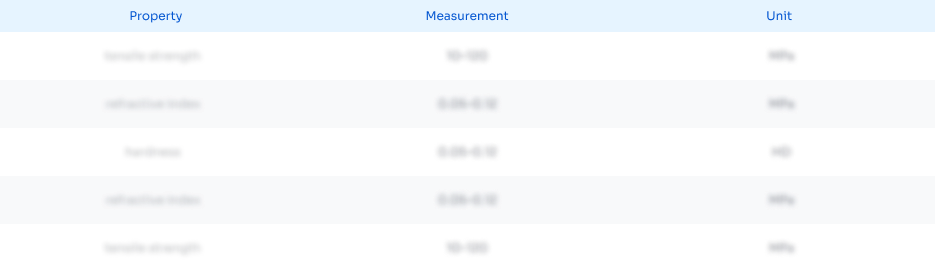
Abstract
Description
Claims
Application Information
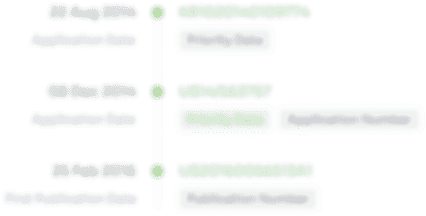
- R&D Engineer
- R&D Manager
- IP Professional
- Industry Leading Data Capabilities
- Powerful AI technology
- Patent DNA Extraction
Browse by: Latest US Patents, China's latest patents, Technical Efficacy Thesaurus, Application Domain, Technology Topic, Popular Technical Reports.
© 2024 PatSnap. All rights reserved.Legal|Privacy policy|Modern Slavery Act Transparency Statement|Sitemap|About US| Contact US: help@patsnap.com