Image super-resolution reconstruction method based on residual convolutional neural network
A convolutional neural network and low-resolution image technology, applied in the field of image processing and computer vision, can solve the problems of shallow network, slow network training speed, gradient disappearance, etc., and achieve the effect of accelerating convergence, avoiding loss, and easy extraction
- Summary
- Abstract
- Description
- Claims
- Application Information
AI Technical Summary
Problems solved by technology
Method used
Image
Examples
Embodiment Construction
[0029] The invention realizes an image super-resolution reconstruction method based on the residual convolutional neural network, improves the residual unit structure of the convolutional neural network, and learns the low-resolution image and the low-resolution image through the connection of multiple residual units. The mapping relationship between high-resolution images is used to reconstruct the high-resolution image using the learned mapping relationship.
[0030] 1 Image preprocessing
[0031] Before network training, the low-resolution image needs to be preprocessed. The specific process is to use the bicubic interpolation method to expand the low-resolution image to the corresponding size image. Then convert the image from RGB space to YCbCr space. Since the human eye is more sensitive to brightness information, only the Y channel of the image is processed and used as the input of the network.
[0032] 2 Feature extraction
[0033] For the input low-resolution image,...
PUM
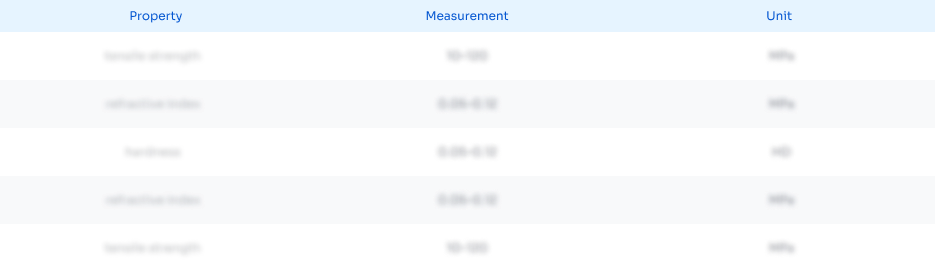
Abstract
Description
Claims
Application Information
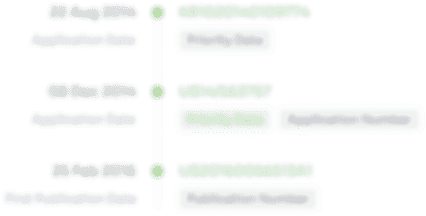
- R&D Engineer
- R&D Manager
- IP Professional
- Industry Leading Data Capabilities
- Powerful AI technology
- Patent DNA Extraction
Browse by: Latest US Patents, China's latest patents, Technical Efficacy Thesaurus, Application Domain, Technology Topic, Popular Technical Reports.
© 2024 PatSnap. All rights reserved.Legal|Privacy policy|Modern Slavery Act Transparency Statement|Sitemap|About US| Contact US: help@patsnap.com