Active learning sample selection strategy integrated with confidence criterion and diversity criterion
A technology of active learning and selection strategies, applied in character and pattern recognition, instruments, biological neural network models, etc., can solve problems such as not considering sample diversity, active learning methods relying on model performance, etc.
- Summary
- Abstract
- Description
- Claims
- Application Information
AI Technical Summary
Problems solved by technology
Method used
Image
Examples
Embodiment Construction
[0033] Specific embodiments of the present invention are described in detail below, but it should be understood that the protection scope of the present invention is not limited by the specific embodiments.
[0034] This embodiment provides an active learning sample selection strategy that combines confidence and diversity criteria, and is applied to a continuous learning framework. The present invention is stated below in conjunction with the examples of the field of audio recognition, and its flow process is as follows figure 1 shown, including the following steps:
[0035] Step 1. Train the model M based on the existing labeled data t .
[0036] First, the strategy is calculated based on the output of the model, we need to use the existing labeled training set D before using this strategy to select samples L build model M t . Among them, the initial training set D L The data of is preprocessed feature data. For example, in the field of audio, the input data of the mo...
PUM
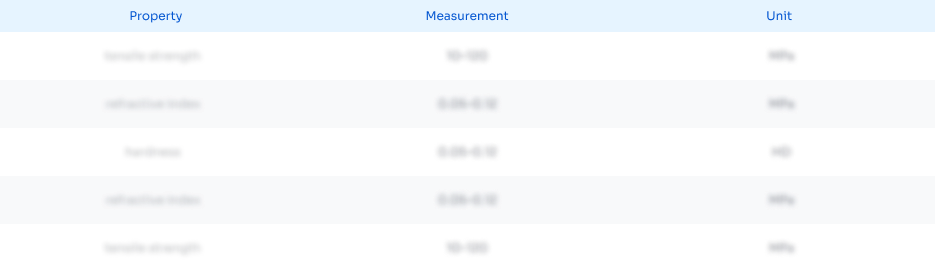
Abstract
Description
Claims
Application Information
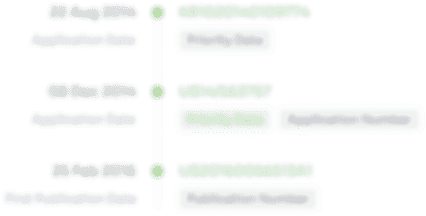
- R&D Engineer
- R&D Manager
- IP Professional
- Industry Leading Data Capabilities
- Powerful AI technology
- Patent DNA Extraction
Browse by: Latest US Patents, China's latest patents, Technical Efficacy Thesaurus, Application Domain, Technology Topic, Popular Technical Reports.
© 2024 PatSnap. All rights reserved.Legal|Privacy policy|Modern Slavery Act Transparency Statement|Sitemap|About US| Contact US: help@patsnap.com