Remotely-supervised Dual-Attention relation classification method and system
A technology of relation classification and remote supervision, applied in special data processing applications, instruments, electrical digital data processing, etc., can solve the problems of model training noise influence, weak performance, high cost ratio, etc., to reduce noisy data, avoid wrong transmission, The effect of improving accuracy
- Summary
- Abstract
- Description
- Claims
- Application Information
AI Technical Summary
Problems solved by technology
Method used
Image
Examples
Embodiment 1
[0054] Such as figure 1 As shown, the embodiment of the present invention provides a remote supervision Dual-Attention relationship classification method, including:
[0055] Align the entity pairs in the knowledge base to the news corpus through remote supervision, and construct an entity pair sentence set;
[0056] The Bi-LSTM model based on the word-level attention mechanism carries out the vector encoding of the word level to the sentence to obtain the semantic feature encoding vector of the sentence;
[0057] The Bi-LSTM model based on the sentence-level attention mechanism encodes and denoises the semantic features of the sentence to obtain the sentence set feature encoding vector;
[0058] The sentence set feature encoding vector and the entity pair translation vector are packaged, and the relationship classification of the entity pair is performed on the obtained package feature.
[0059] Such as figure 2 Shown is a specific flow chart of the embodiment of the pres...
Embodiment 2
[0081] Based on the same inventive concept, the present invention also provides a remote-supervised Dual-Attention relationship classification system, including:
[0082] Building blocks for aligning entity pairs in the knowledge base to news corpus through remote supervision, and constructing entity-pair sentence collections;
[0083] The first vector module is used to carry out the vector encoding of the word level to the sentence based on the Bi-LSTM model of the word level attention mechanism, to obtain the semantic feature encoding vector of the sentence;
[0084] The second vector module is used to encode and denoise the semantic features of the sentence based on the Bi-LSTM model of the sentence-level attention mechanism to obtain a sentence set feature encoding vector;
[0085] The relationship classification module is used to package the sentence set feature encoding vector and the entity pair translation vector, and perform entity pair relationship classification on ...
Embodiment 3
[0105] In the knowledge base WikiData, it is known that the entity pair "Jack Ma", "Alibaba" and the corresponding relationship set "founder", "CEO" and other relationships are classified from the Internet data into the entities containing the entity pair "Jack Ma" and "Alibaba". Several sentences, here are examples of sentences in which four entities co-occur.
[0106] Sentence 1: “Female executives are Alibaba’s secret sauce, founder Jack Masays.”
[0107] Sentence 2: "At a conference hosted by All Things D last week, Alibaba CEO Jack Ma said that he was interested in Yahoo."
[0108] Sentence 3: “Internet entrepreneur Jack Ma started a Chinese version of the Yellow Pages that was Alibaba’s precursor in Hanzhou, China.”
[0109] Sentence 4: "Alibaba has brought more small U.S. businesses onto the company's sites, but this is the first time Ma has discussed specific targets."
[0110] Sentences 3 and 4 do not express the predefined relationship of the knowledge base. One o...
PUM
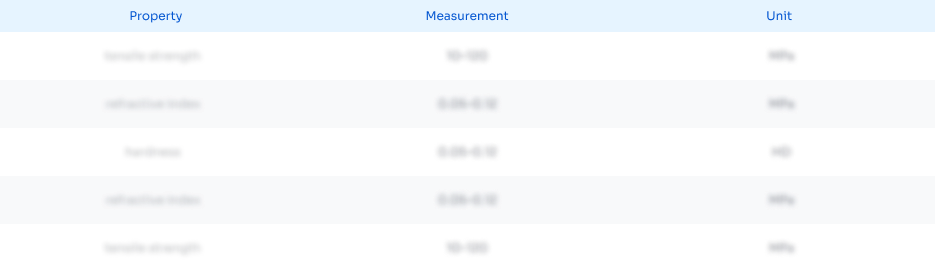
Abstract
Description
Claims
Application Information
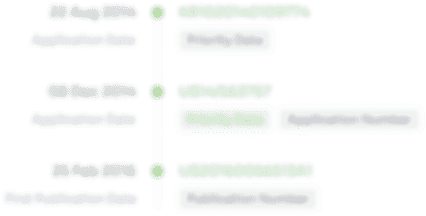
- R&D Engineer
- R&D Manager
- IP Professional
- Industry Leading Data Capabilities
- Powerful AI technology
- Patent DNA Extraction
Browse by: Latest US Patents, China's latest patents, Technical Efficacy Thesaurus, Application Domain, Technology Topic, Popular Technical Reports.
© 2024 PatSnap. All rights reserved.Legal|Privacy policy|Modern Slavery Act Transparency Statement|Sitemap|About US| Contact US: help@patsnap.com