A remote-supervised dual-attention relationship classification method and system
A technology of relational classification and remote supervision, applied in text database clustering/classification, unstructured text data retrieval, instruments, etc., can solve the problems of model training noise influence, weak performance, high cost ratio, etc., to reduce noise data, Avoid mistransmission and improve accuracy
- Summary
- Abstract
- Description
- Claims
- Application Information
AI Technical Summary
Problems solved by technology
Method used
Image
Examples
Embodiment 1
[0054] like figure 1 As shown, an embodiment of the present invention provides a remotely supervised Dual-Attention relationship classification method, including:
[0055] Align entity pairs in the knowledge base to the news corpus through remote supervision to construct entity pair sentence sets;
[0056] The Bi-LSTM model based on the word-level attention mechanism performs the word-level vector encoding on the sentence to obtain the semantic feature encoding vector of the sentence;
[0057] The Bi-LSTM model based on the sentence-level attention mechanism encodes and denoises the semantic features of the sentence, and obtains the feature encoding vector of the sentence set;
[0058] The sentence set feature encoding vector and the entity pair translation vector are packaged, and the obtained package features are classified into entity pairs.
[0059] like figure 2 Shown is a specific flow chart of an embodiment of the present invention.
[0060] Preferably, by aligning...
Embodiment 2
[0081] Based on the same inventive concept, the present invention also provides a remotely supervised Dual-Attention relation classification system, including:
[0082] Building module for aligning entity pairs in the knowledge base to news corpus through remote supervision, and constructing entity pair sentence sets;
[0083] The first vector module is used to perform word-level vector encoding on the sentence based on the Bi-LSTM model of the word-level attention mechanism to obtain a semantic feature encoding vector of the sentence;
[0084] The second vector module is used for encoding and denoising the semantic features of the sentence based on the Bi-LSTM model of the sentence-level attention mechanism to obtain a sentence set feature encoding vector;
[0085] The relationship classification module is used to package the sentence set feature encoding vector and the entity pair translation vector, and perform the relationship classification of the entity pair on the obtai...
Embodiment 3
[0105] In the knowledge base WikiData, the entity pairs "Jack Ma", "Alibaba" and the corresponding relationship sets "founder", "CEO" and other relationships are known, and the Internet data is classified into the entity pairs "Jack Ma" and "Alibaba". Several sentences, here are examples of sentences in which four entities co-occur.
[0106] Sentence 1: "Female executives are Alibaba's secret sauce, founder Jack Masays."
[0107] Sentence 2: "At a conference hosted by All Things D last week, Alibaba CEO Jack Ma said that he was interested in Yahoo."
[0108] Sentence 3: "Internet entrepreneur Jack Ma started a Chinese version of the Yellow Pages that was Alibaba's precursor in Hanzhou, China."
[0109] Sentence 4: "Alibaba has brought more small U.S. businesses onto the company's sites, but this is the first time Ma has discussed specific targets."
[0110] Sentences 3 and 4 do not express the predefined relationship of the knowledge base. One of the purposes of the present...
PUM
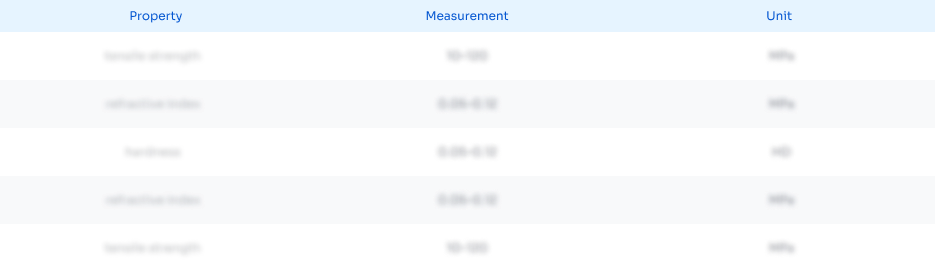
Abstract
Description
Claims
Application Information
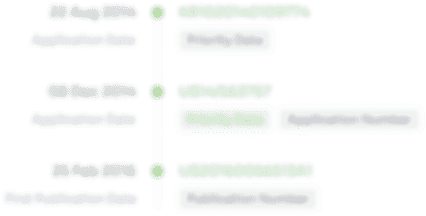
- R&D Engineer
- R&D Manager
- IP Professional
- Industry Leading Data Capabilities
- Powerful AI technology
- Patent DNA Extraction
Browse by: Latest US Patents, China's latest patents, Technical Efficacy Thesaurus, Application Domain, Technology Topic.
© 2024 PatSnap. All rights reserved.Legal|Privacy policy|Modern Slavery Act Transparency Statement|Sitemap