Method for improving video resolution through convolutional neural network
A convolutional neural network and resolution technology, which is applied in the field of improving video resolution by using convolutional neural network, can solve problems such as unsatisfactory high-resolution video effects, underutilization, and complex algorithm calculations, etc., to reduce local maximum Optimal Risk, Increased Training Speed, Effects of Accelerated Speed
- Summary
- Abstract
- Description
- Claims
- Application Information
AI Technical Summary
Problems solved by technology
Method used
Image
Examples
Embodiment Construction
[0032] The technical solution of the present invention will be further described below in conjunction with the accompanying drawings.
[0033] The present invention builds a video super-resolution reconstruction model based on a convolutional neural network for video image sequences, and sets the hyperparameters of the convolutional neural network based on the characteristics of the video image sequence during the model building process, including convolution kernel size, The number of layers, etc.; then use the image training weights generated by the super-resolution method of a single image to initialize the weight parameters of the video super-resolution reconstruction model, and make full use of the redundant information between video frames to use multi-frame video images as convolution The input of the neural network model, through an incremental iterative method, finally obtains a high-resolution video. refer to figure 1, the video super-resolution method proposed by t...
PUM
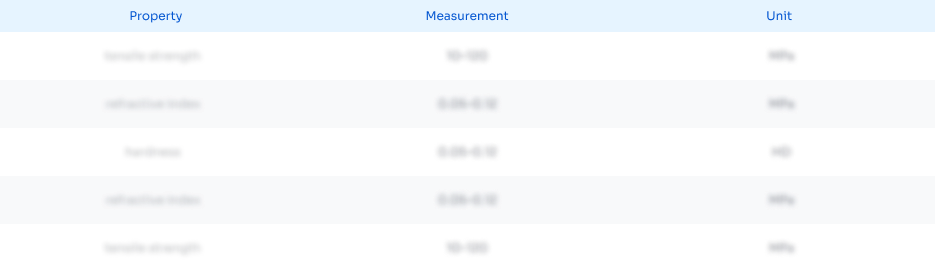
Abstract
Description
Claims
Application Information
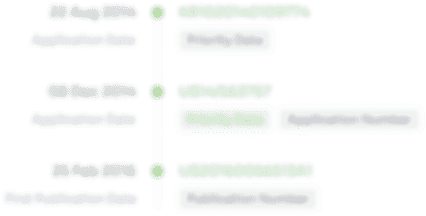
- R&D Engineer
- R&D Manager
- IP Professional
- Industry Leading Data Capabilities
- Powerful AI technology
- Patent DNA Extraction
Browse by: Latest US Patents, China's latest patents, Technical Efficacy Thesaurus, Application Domain, Technology Topic, Popular Technical Reports.
© 2024 PatSnap. All rights reserved.Legal|Privacy policy|Modern Slavery Act Transparency Statement|Sitemap|About US| Contact US: help@patsnap.com