Network flow type prediction method based on deep learning
A technology of deep learning and prediction methods, applied in the field of network flow type prediction based on deep learning, can solve the problem of ignoring the real demand of duration resources, and achieve the effect of improving prediction accuracy, low overhead, and improving prediction accuracy.
- Summary
- Abstract
- Description
- Claims
- Application Information
AI Technical Summary
Problems solved by technology
Method used
Image
Examples
Embodiment
[0040] The present invention uses deep learning to analyze the joint characteristics of four dimensional data, such as the time distribution characteristics of the flow, the real-time size characteristics of the flow, the message header characteristics, and the socket characteristics, so as to realize the prediction of the flow type. The basic principles are as follows:
[0041] Based on the SDN (Software Defined Network) architecture, the multi-level prediction scheme of "edge pre-classification + central fine classification" is adopted, that is, the SDN switches and controllers at the edge of the network are respectively constructed for pre-classification and fine classification. deep learning model. In the pre-classification stage, the computing resources and links of each switch in the SDN network are used to build a distributed deep learning network, in which each switch contributes a small part of resources to realize the computing functions of several neurons, and the ne...
PUM
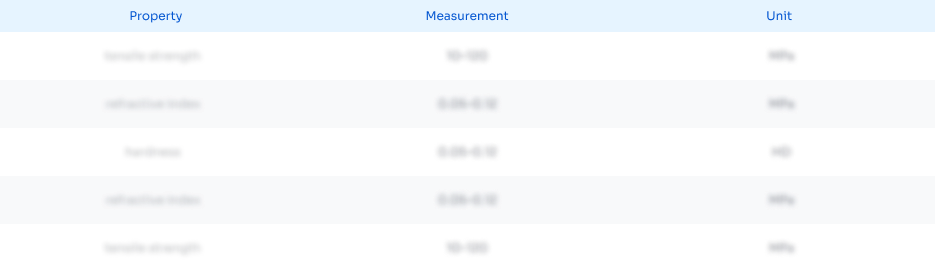
Abstract
Description
Claims
Application Information
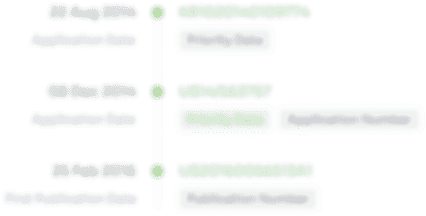
- R&D Engineer
- R&D Manager
- IP Professional
- Industry Leading Data Capabilities
- Powerful AI technology
- Patent DNA Extraction
Browse by: Latest US Patents, China's latest patents, Technical Efficacy Thesaurus, Application Domain, Technology Topic, Popular Technical Reports.
© 2024 PatSnap. All rights reserved.Legal|Privacy policy|Modern Slavery Act Transparency Statement|Sitemap|About US| Contact US: help@patsnap.com