Power grid load prediction method based on depth LSTM neural network
A power grid load and neural network technology, applied in forecasting, instrumentation, data processing applications, etc., can solve the problems of low power grid load forecasting accuracy and short time steps, so as to avoid calculation and time-consuming forecasting, improve real-time performance, The effect of ensuring integrity
- Summary
- Abstract
- Description
- Claims
- Application Information
AI Technical Summary
Problems solved by technology
Method used
Image
Examples
Embodiment Construction
[0036] Embodiments of the present invention are described in detail below, examples of which are shown in the drawings, wherein the same or similar reference numerals designate the same or similar elements or elements having the same or similar functions throughout. The embodiments described below by referring to the figures are exemplary and are intended to explain the present invention and should not be construed as limiting the present invention.
[0037] The power grid load forecasting method based on the deep LSTM neural network according to the embodiment of the present invention will be described below with reference to the accompanying drawings.
[0038] figure 1 It is a flowchart of a power grid load forecasting method based on a deep LSTM neural network according to an embodiment of the present invention.
[0039] Such as figure 1 As shown, the power grid load forecasting method based on the deep LSTM neural network of the embodiment of the present invention includ...
PUM
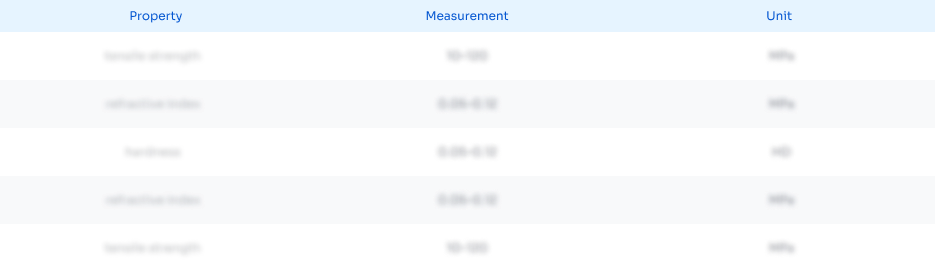
Abstract
Description
Claims
Application Information
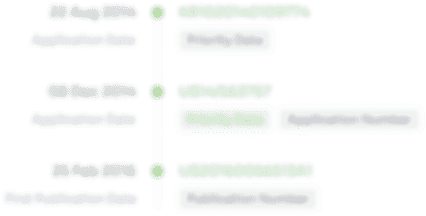
- R&D
- Intellectual Property
- Life Sciences
- Materials
- Tech Scout
- Unparalleled Data Quality
- Higher Quality Content
- 60% Fewer Hallucinations
Browse by: Latest US Patents, China's latest patents, Technical Efficacy Thesaurus, Application Domain, Technology Topic, Popular Technical Reports.
© 2025 PatSnap. All rights reserved.Legal|Privacy policy|Modern Slavery Act Transparency Statement|Sitemap|About US| Contact US: help@patsnap.com