Word embedding-based deep zero sample classification method
A classification method and sample technology, applied in the field of pattern recognition, can solve problems such as low efficiency and consumption of manpower and material resources, and achieve the effect of improving efficiency and human interference factors
- Summary
- Abstract
- Description
- Claims
- Application Information
AI Technical Summary
Problems solved by technology
Method used
Image
Examples
Embodiment Construction
[0032] Below in conjunction with accompanying drawing, technical scheme of the present invention is described in further detail:
[0033] Those skilled in the art can understand that, unless otherwise defined, all terms (including technical terms and scientific terms) used herein have the same meaning as commonly understood by one of ordinary skill in the art to which this invention belongs. It should also be understood that terms such as those defined in commonly used dictionaries should be understood to have a meaning consistent with the meaning in the context of the prior art, and will not be interpreted in an idealized or overly formal sense unless defined as herein Explanation.
[0034] The present invention provides a deep zero-sample image learning algorithm based on a word embedding model, which realizes zero-sample classification of image features by combining text features, including the following steps:
[0035] Step 1, input image training set x i Represents th...
PUM
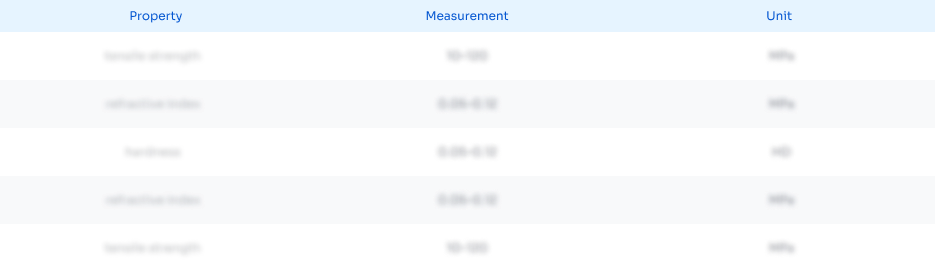
Abstract
Description
Claims
Application Information
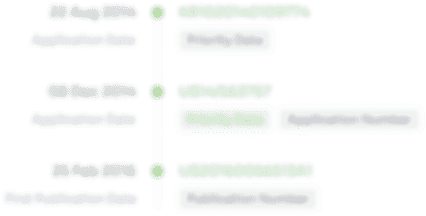
- R&D Engineer
- R&D Manager
- IP Professional
- Industry Leading Data Capabilities
- Powerful AI technology
- Patent DNA Extraction
Browse by: Latest US Patents, China's latest patents, Technical Efficacy Thesaurus, Application Domain, Technology Topic, Popular Technical Reports.
© 2024 PatSnap. All rights reserved.Legal|Privacy policy|Modern Slavery Act Transparency Statement|Sitemap|About US| Contact US: help@patsnap.com