Mura defect level judgment method and device based on deep learning
A technology of defect level and deep learning, which is applied in the direction of optical testing defects/defects, measuring devices, scientific instruments, etc., can solve problems such as low contrast, uneven overall brightness, blurred edges, etc., to reduce the interference of complex backgrounds and artificial Cost and time cost, effect of improving accuracy
- Summary
- Abstract
- Description
- Claims
- Application Information
AI Technical Summary
Problems solved by technology
Method used
Image
Examples
Embodiment
[0044] The present invention uses the AlexNet model to classify the Mura defects, and the AlexNet includes eight weighted layers; the first five layers are convolutional layers, and the remaining three layers are fully connected layers. The output of the last fully connected layer is fed to a 1000-way softmax layer, which produces a distribution covering 1000 class labels. AlexNet maximizes the multi-class Logistic regression objective, which is equivalent to maximizing the average log probability of the correct label in the training samples under the prediction distribution.
[0045] At present, mura defects are generally divided into C K L Y N 5 categories. The mura defect image is first normalized into a 224×224 image, which enters the network as an input neuron. After the first layer of convolution, the original convolution data is obtained, and a ReLU is performed first. And Norm transformation, and then perform pooling, and pass it to the next layer as the output; after ...
PUM
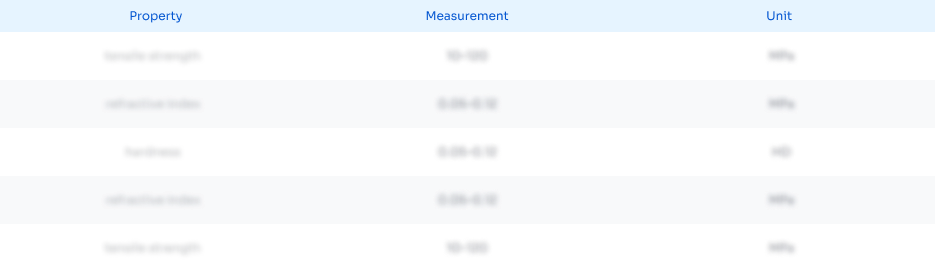
Abstract
Description
Claims
Application Information
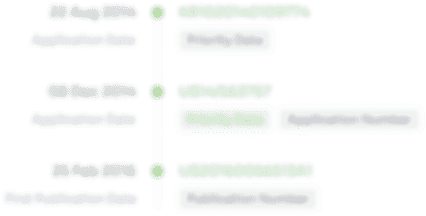
- R&D Engineer
- R&D Manager
- IP Professional
- Industry Leading Data Capabilities
- Powerful AI technology
- Patent DNA Extraction
Browse by: Latest US Patents, China's latest patents, Technical Efficacy Thesaurus, Application Domain, Technology Topic, Popular Technical Reports.
© 2024 PatSnap. All rights reserved.Legal|Privacy policy|Modern Slavery Act Transparency Statement|Sitemap|About US| Contact US: help@patsnap.com