Multi-objective deep convolution generative adversarial network model and learning method thereof
A deep convolution and network model technology, applied in the field of deep learning, can solve problems such as crash mode and instability
- Summary
- Abstract
- Description
- Claims
- Application Information
AI Technical Summary
Problems solved by technology
Method used
Image
Examples
Embodiment Construction
[0087] Below in conjunction with accompanying drawing, implementation steps and experimental effects of the present invention are described in further detail:
[0088] refer to figure 1 , the specific implementation steps of the present invention are as follows:
[0089] Step 1, population initialization.
[0090] Initialize n generators by random generation and n discriminator parameters And combined into n groups of generative confrontation networks in order:
[0091] Enter the local search stage to learn and optimize each group of generative confrontation networks.
[0092] Set the structure of the generator G in DCGAN: a 5-layer micro-step convolutional neural network composed of input layer→deconvolution layer→deconvolution layer→deconvolution layer→deconvolution layer→output layer, given The feature map of each layer, and determine the size of each deconvolution layer and initialize the weights and biases randomly. The parameters of each layer are set as follows...
PUM
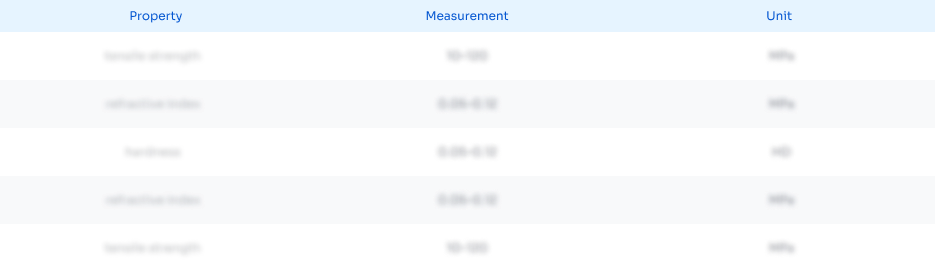
Abstract
Description
Claims
Application Information
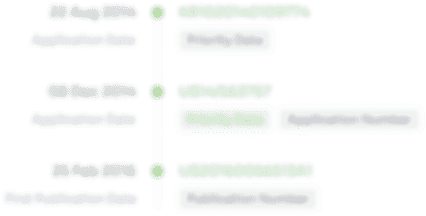
- R&D Engineer
- R&D Manager
- IP Professional
- Industry Leading Data Capabilities
- Powerful AI technology
- Patent DNA Extraction
Browse by: Latest US Patents, China's latest patents, Technical Efficacy Thesaurus, Application Domain, Technology Topic, Popular Technical Reports.
© 2024 PatSnap. All rights reserved.Legal|Privacy policy|Modern Slavery Act Transparency Statement|Sitemap|About US| Contact US: help@patsnap.com