Vehicle recognition and tracking method based on convolutional neural networks
A convolutional neural network and vehicle recognition technology, which is applied in biological neural network models, neural architectures, character and pattern recognition, etc., can solve complex scenes and tricky angles that are difficult to apply, difficult to meet time characteristic requirements, and applicable to a single scene and other problems, to achieve the effect of being easy to realize by computer, saving calculation overhead, and having strong nonlinear mapping ability
- Summary
- Abstract
- Description
- Claims
- Application Information
AI Technical Summary
Problems solved by technology
Method used
Image
Examples
Embodiment 1
[0041] In the existing tracking and recognition technology, the method of template matching is used to process the data very fast, but the detailed features in the class are often not accurately distinguished, and classification errors are prone to occur; while the binary classification method needs to select a suitable Features, and a classifier model is trained through a large number of training pictures. The detection results are often more accurate, but sufficient preparation is required, and it is difficult to meet the time characteristic requirements during the tracking process.
[0042] At present, the recognition and tracking of objects in surveillance video images is of great significance for traffic congestion mitigation, vehicle speed measurement, and emergency traffic incident handling. To deal with the above traffic problems, it is necessary to adopt relatively fast and accurate tracking and identification technology. However, in terms of time characteristics and ...
Embodiment 2
[0069] The vehicle recognition and tracking method based on the convolutional neural network is the same as embodiment 1, the construction of the fast regional convolutional neural network described in the step (1b) of the present invention, see figure 2 , The structure of the fast regional convolutional neural network is, in turn, convolutional layer conv1, pooling layer pool1, convolutional layer conv2, pooling layer pool2, convolutional layer conv3, pooling layer pool3, convolutional layer conv4, pooling layer pool4, convolutional layer conv5, convolutional layer rpn_conv, convolutional layer rpn_cls_score, convolutional layer rpn_bbox_pred, region of interest pooling layer roi_pool, fully connected layer fc6, fully connected layer fc7, fully connected layer fc8, classification layer cls_prob, coordinates Layer bbox_pred.
Embodiment 3
[0071] The vehicle recognition and tracking method based on convolutional neural network is the same as embodiment 1-2, and the steps of the fast regional convolutional neural network constructed as described in step (1b) are as follows:
[0072] (1b.1), input a monitoring image of any size into the convolution layer conv1, use 64 convolution kernels, perform a convolution operation with a block size of 3×3 pixels and a step size of 1 pixel, and obtain 64 channels feature map;
[0073] (1b.2), input the 64-channel feature map output by the convolutional layer conv1 to the pooling layer pool1 to obtain a 64-channel feature map;
[0074] (1b.3), input the 64-channel feature map output by the pooling layer pool1 to the convolutional layer conv2, and use 128 convolution kernels to perform convolution with a block size of 3×3 pixels and a step size of 1 pixel Operation, get 128 channel feature map;
[0075] (1b.4), input the 128-channel feature map output by the convolutional lay...
PUM
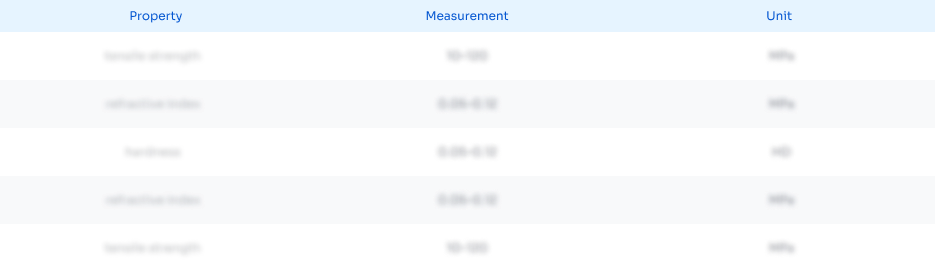
Abstract
Description
Claims
Application Information
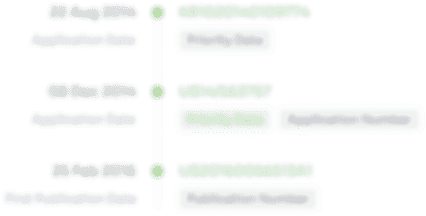
- R&D Engineer
- R&D Manager
- IP Professional
- Industry Leading Data Capabilities
- Powerful AI technology
- Patent DNA Extraction
Browse by: Latest US Patents, China's latest patents, Technical Efficacy Thesaurus, Application Domain, Technology Topic, Popular Technical Reports.
© 2024 PatSnap. All rights reserved.Legal|Privacy policy|Modern Slavery Act Transparency Statement|Sitemap|About US| Contact US: help@patsnap.com