Learning type image processing method, system and server
An image processing and learning technology, applied in the field of image processing, can solve the problems of simple samples not being optimized, increase the intra-class distance of most simple samples, etc., and achieve the effects of good robustness, accurate classification results, and high degree of convergence
- Summary
- Abstract
- Description
- Claims
- Application Information
AI Technical Summary
Problems solved by technology
Method used
Image
Examples
Embodiment
[0054] It should be pointed out that the basic structure of the convolutional neural network includes two layers, one is the feature extraction layer, the input of each neuron is connected to the local receptive field of the previous layer, and the local features are extracted. Once the local feature is extracted, the positional relationship between it and other features is also determined; the second is the feature map layer, each calculation layer of the network is composed of multiple feature maps, each feature map is a plane, All neurons on the plane have equal weights. The feature map structure uses the sigmoid function with a small influence function kernel as the activation function of the convolutional network, so that the feature map has displacement invariance. In addition, since neurons on a mapping plane share weights, the number of free parameters of the network is reduced. Each convolutional layer in the convolutional neural network is followed by a calculation ...
PUM
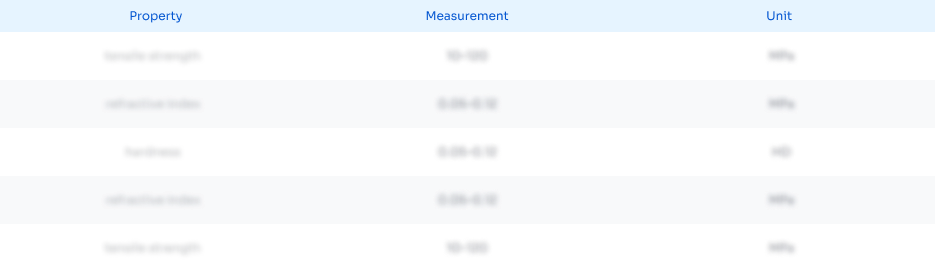
Abstract
Description
Claims
Application Information
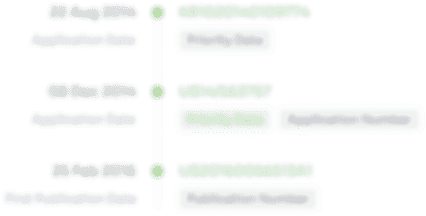
- R&D Engineer
- R&D Manager
- IP Professional
- Industry Leading Data Capabilities
- Powerful AI technology
- Patent DNA Extraction
Browse by: Latest US Patents, China's latest patents, Technical Efficacy Thesaurus, Application Domain, Technology Topic, Popular Technical Reports.
© 2024 PatSnap. All rights reserved.Legal|Privacy policy|Modern Slavery Act Transparency Statement|Sitemap|About US| Contact US: help@patsnap.com