Confrontation migration learning method and system for cross-border big data analysis
A technology of transfer learning and big data, applied in the field of data analysis, it can solve the problems that deep neural network is difficult to capture data distribution characteristics, large data distribution offset, data distribution offset, etc., so as to reduce the amount of data calculation and distribution offset. Reduce and avoid the effect of data distribution shift
- Summary
- Abstract
- Description
- Claims
- Application Information
AI Technical Summary
Problems solved by technology
Method used
Image
Examples
Embodiment Construction
[0023] The specific implementation manners of the present invention will be further described in detail below in conjunction with the accompanying drawings and embodiments. The following examples are used to illustrate the present invention, but are not intended to limit the scope of the present invention.
[0024] Such as figure 1 As shown, the embodiment of the present invention provides an adversarial transfer learning method for cross-border big data analysis, the method includes:
[0025] Step 1, input the respective unlabeled data sets of the source domain and the target domain into the preset deep neural network and propagate forward to obtain the tensor sets corresponding to the respective unlabeled data sets of the source domain and the target domain; the tensor sets The tensor in the quantity set is the tensor product of data vectors of all data layers in the preset data layer set in the preset deep neural network when the corresponding unlabeled data is used as input...
PUM
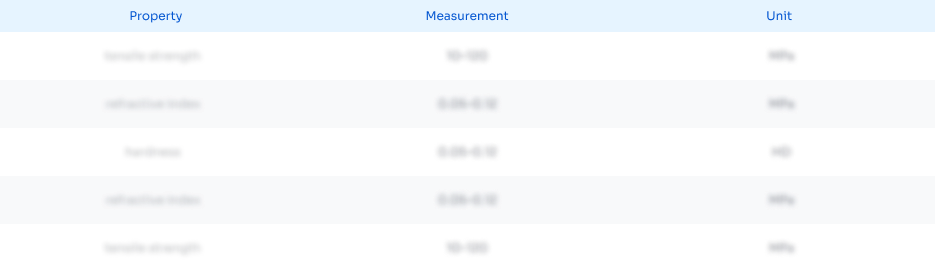
Abstract
Description
Claims
Application Information
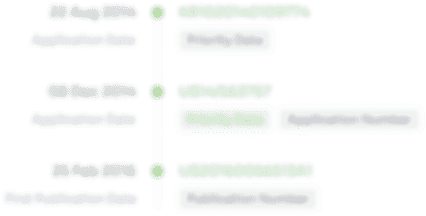
- R&D
- Intellectual Property
- Life Sciences
- Materials
- Tech Scout
- Unparalleled Data Quality
- Higher Quality Content
- 60% Fewer Hallucinations
Browse by: Latest US Patents, China's latest patents, Technical Efficacy Thesaurus, Application Domain, Technology Topic, Popular Technical Reports.
© 2025 PatSnap. All rights reserved.Legal|Privacy policy|Modern Slavery Act Transparency Statement|Sitemap|About US| Contact US: help@patsnap.com