Accurate molecular deconvolution of mixtures samples
A sample, fetal technique for precise molecular deconvolution of mixture samples
- Summary
- Abstract
- Description
- Claims
- Application Information
AI Technical Summary
Problems solved by technology
Method used
Image
Examples
Embodiment 1
[0109] Example 1: Maximizing the Enumeration Effect by HaploSeq in the Context of NIPT
[0110] The method described here takes full advantage of HaploSeq-based cross-chromosomal parental haplotypes through long-range and maximized enumeration, thus facilitating minimal and low-depth MPcfDNA sequencing. This innovative approach supports figure 1 The theoretical limit estimate shown in A: the long parental haplotype significantly reduces the required MPcfDNA sequencing depth to determine the fetal genome ( figure 2 B(i)). Furthermore, the availability of parental haplotypes across chromosome-groups (e.g. exome) by HaploSeq means that fetal-group (e.g. exome) can be determined from group-targeted sequencing of MPcfDNA ( figure 2 B(ii))—The key is that the -group elements are distributed throughout the chromosome, thus, cross-chromosomal haplotypes of parental-groups enable maximum enumeration and minimize MPcfDNA-group sequencing. Furthermore, any targeted fetal loci can be...
Embodiment 2
[0113] Example 2: HMM with enumerated input from low-depth sequencing of MPcfDNA accurately determines fetal sequence
[0114] Using the enumerated allele fractions as observations, one aspect of the present disclosure is to use an HMM algorithm that assumes the hidden haplotype state of the fetus (2-state: M1, M2) as determined by MHet-PeqMT et al. A binomial distribution among the alleles truly emits the enumerated allele fractions. The 2-state binomial HMM model identified fetal genetic content with >98% accuracy in 2X simulated MPcfDNA sequencing with 5Mb enumeration ( Figure 4 A). This is remarkable because the method achieves comparable accuracy to other methods using >40X MPcfDNA sequencing, but at 2X MPcfDNA sequencing, thus reducing the sequencing cost by more than 20-fold. While the binomial HMM algorithm is a natural choice because it directly models the probability of emitting the hidden state of an enumerated observation via the binomial expectation (p(success)...
Embodiment 3
[0115] Example 3: Optimization of enumeration window size
[0116] An important feature of the present disclosure is the correct parental haplotype enumeration block window. The 5Mb sliding window provided a robust input for a 2-state Gaussian HMM to predict fetal genotypes and haplotypes with >99% accuracy in our simulated MPcfDNA data of 2X sequenced MHet-PeqMT alleles ( Figure 4 A). A longer enumeration window size enables a more robust cumulative input to the HMM, but also a higher probability of recombination within that window. Meanwhile, shorter window sizes may not have enough data points to generate robust HMM inputs. Therefore, to address this tradeoff, multiple window sizes were analyzed to utilize the best possible HMM input to obtain the highest accuracy for fetal sequence inference. Our analysis of the MHet-PeqMT alleles revealed that enumeration within a ~2 Mb window size upstream and downstream of each MHet-PeqMT allele yielded the best accuracy (~99.5%) fo...
PUM
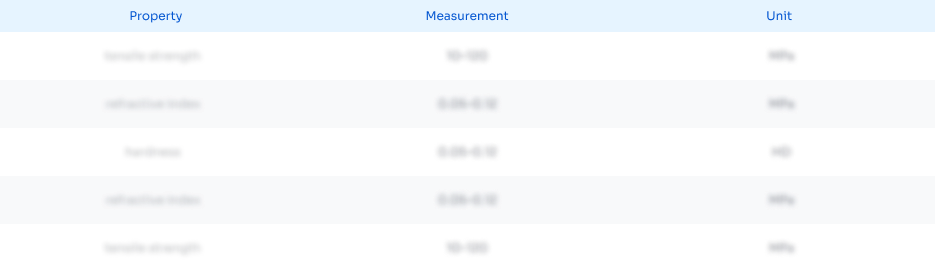
Abstract
Description
Claims
Application Information
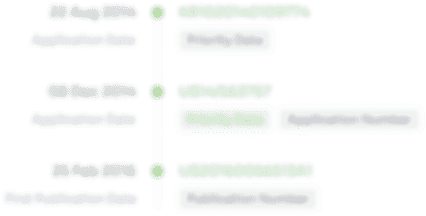
- R&D Engineer
- R&D Manager
- IP Professional
- Industry Leading Data Capabilities
- Powerful AI technology
- Patent DNA Extraction
Browse by: Latest US Patents, China's latest patents, Technical Efficacy Thesaurus, Application Domain, Technology Topic, Popular Technical Reports.
© 2024 PatSnap. All rights reserved.Legal|Privacy policy|Modern Slavery Act Transparency Statement|Sitemap|About US| Contact US: help@patsnap.com