Glowworm swarm optimization method-based image multi-threshold segmentation method
A firefly, multi-threshold technology, applied in the field of image processing, can solve the problems of long time consumption and low segmentation accuracy, and achieve the effect of fast segmentation and high segmentation threshold accuracy.
- Summary
- Abstract
- Description
- Claims
- Application Information
AI Technical Summary
Problems solved by technology
Method used
Image
Examples
Embodiment 1
[0053] Taking a color image of the MSRA salient target data set as an example, the number is 1_56_56055, the steps of the image multi-threshold method based on the firefly swarm optimization method are as follows:
[0054] (1) Image preprocessing and determining the number of image thresholds
[0055] figure 1 A flowchart of this embodiment is given. exist figure 1 , read in the image and convert it to grayscale to get a grayscale image, see figure 2 , perform median filter processing, denoising processing, and obtain a grayscale histogram, see image 3 ,exist image 3 The middle abscissa is the gray value, and the ordinate is the number of pixels. Points (9, 741), points (82, 1511), and points (118, 2953) are peaks, and the height of the peaks is higher than the baseline 738. Determine The number of peaks is 3, and the number D of the obtained image threshold is 2; the grayscale image is processed by a first-level wavelet transform, and the obtained grayscale image is t...
Embodiment 2
[0087] Taking a color image of the MSRA salient target data set as an example, the number is 1_56_56055, the steps of the image multi-threshold method based on the firefly swarm optimization method are as follows:
[0088] In this embodiment, steps (1) and (2) are the same as in Embodiment 1.
[0089] In the parameter setting step 1) of step (3), the maximum number of iterations M of the firefly swarm optimization method is 100, the iteration count m is 1-100, the population size G of fireflies is 50, and the firefly count i is 1-50, The firefly count j is 1-50 and j≠i, the step size S is 15, the number of fireflies in the neighborhood α is 42, the neighborhood change rate β is 0.5, the fluorescein volatilization factor h is 0.8, and the fitness function extraction ratio γ is 0.8, the maximum decision radius r max is 140, the initial fluorescence brightness L of fireflies 0 30, the initial induction radius r 0 is 70, and the firefly decision radius is r i , the position of...
Embodiment 3
[0092] Taking a color image of the MSRA salient target data set as an example, the number is 1_56_56055, the steps of the image multi-threshold method based on the firefly swarm optimization method are as follows:
[0093] In this embodiment, steps (1) and (2) are the same as in Embodiment 1.
[0094] In the parameter setting step 1) of step (3), the maximum number of iterations M of the firefly swarm optimization method is 300, the iteration count m is 1-300, the population size G of fireflies is 150, and the firefly count i is 1-150, The firefly count j is 1-150 and j≠i, the step size S is 17, the number of fireflies in the neighborhood α is 49, the neighborhood change rate β is 0.9, the fluorescein volatilization factor h is 0.99, and the fitness function extraction ratio γ is 0.99, the maximum decision radius r max is 150, the initial fluorescence brightness L of fireflies 0 35, the initial induction radius r 0 is 75, and the firefly decision radius is r i , the positi...
PUM
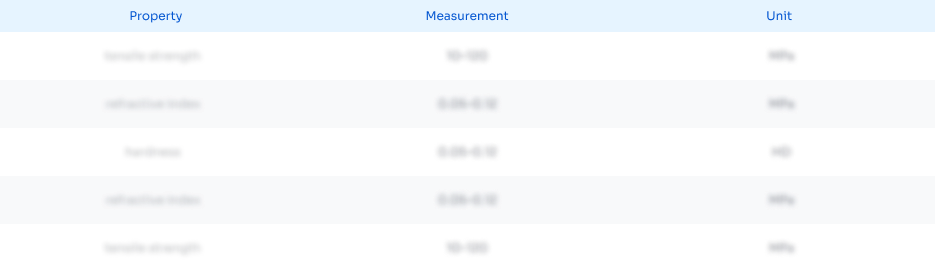
Abstract
Description
Claims
Application Information
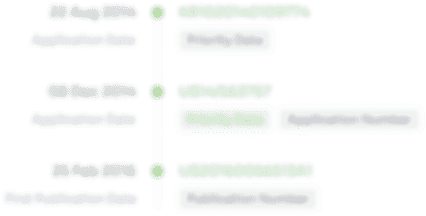
- R&D Engineer
- R&D Manager
- IP Professional
- Industry Leading Data Capabilities
- Powerful AI technology
- Patent DNA Extraction
Browse by: Latest US Patents, China's latest patents, Technical Efficacy Thesaurus, Application Domain, Technology Topic, Popular Technical Reports.
© 2024 PatSnap. All rights reserved.Legal|Privacy policy|Modern Slavery Act Transparency Statement|Sitemap|About US| Contact US: help@patsnap.com