Deep learning-based super-resolution image reconstruction method and system
A super-resolution and image reconstruction technology, applied in image data processing, graphic image conversion, instruments, etc., can solve the problems of loss of high-frequency details, lack of correlation of frequency-domain data, and excessively smooth images, so as to achieve sufficient high-frequency details. , Improve the resolution, the effect of high-resolution images is clear
- Summary
- Abstract
- Description
- Claims
- Application Information
AI Technical Summary
Problems solved by technology
Method used
Image
Examples
Embodiment Construction
[0048] In order to enable those skilled in the art to better understand the technical solutions of the present invention, the present invention will be further described in detail below in conjunction with the accompanying drawings.
[0049] Such as figure 1 As shown, a deep learning-based super-resolution image reconstruction method provided by an embodiment of the present invention includes the following steps:
[0050] S101. Acquiring images to be reconstructed and training data;
[0051] The picture to be reconstructed refers to the super-resolution picture that needs to be reconstructed through a multi-layer convolutional neural network; the training data refers to pictures of various resolutions required for deep learning; each picture corresponds to a picture group, and the picture group It includes a high-resolution picture and at least one low-resolution picture corresponding thereto.
[0052] Such as figure 2 As shown, in step S101, the acquisition of the trainin...
PUM
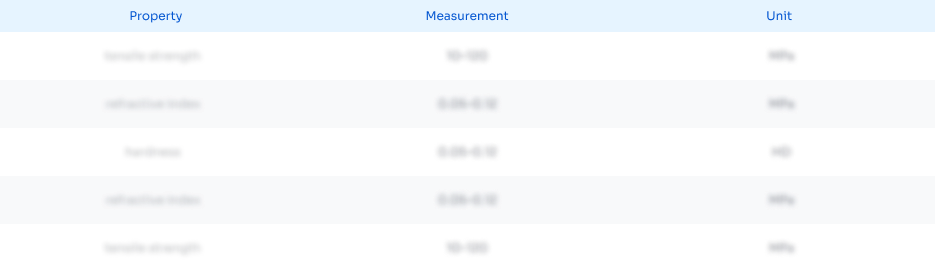
Abstract
Description
Claims
Application Information
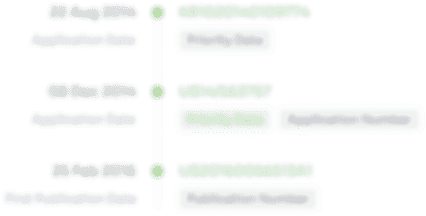
- R&D Engineer
- R&D Manager
- IP Professional
- Industry Leading Data Capabilities
- Powerful AI technology
- Patent DNA Extraction
Browse by: Latest US Patents, China's latest patents, Technical Efficacy Thesaurus, Application Domain, Technology Topic, Popular Technical Reports.
© 2024 PatSnap. All rights reserved.Legal|Privacy policy|Modern Slavery Act Transparency Statement|Sitemap|About US| Contact US: help@patsnap.com