A Dynamic Adjustment Method Based on DCGAN Performance
A dynamic adjustment and performance technology, applied in neural learning methods, neural architectures, biological neural network models, etc., can solve problems such as network model collapse, discriminator ability improvement limitations, small gradient values, etc., to ensure robustness and avoid patterns Crash, robust effects
- Summary
- Abstract
- Description
- Claims
- Application Information
AI Technical Summary
Problems solved by technology
Method used
Image
Examples
Embodiment
[0038] This embodiment discloses a dynamic adjustment method based on DCGAN performance, which specifically includes the following steps:
[0039] Step S1, using the deep convolutional neural network CNN as the generative confrontation network GAN of the generator and discriminator, and constructing the deep convolutional generative confrontation network DCGAN.
[0040] In the GAN framework proposed by GoodFellow, any differentiable function can function as a generator and a discriminator. The generative confrontation network GAN using the convolutional neural network CNN as the generator and the discriminator is DCGAN. attached by figure 1 It can be seen that the image is input to the generator, and after a series of transposed convolutions, it gradually changes from low-dimensional to high-dimensional, and finally outputs an image that conforms to the dimension. Throughout the process, nonlinear layers and batch normalization are applied after each neural network layer.
...
PUM
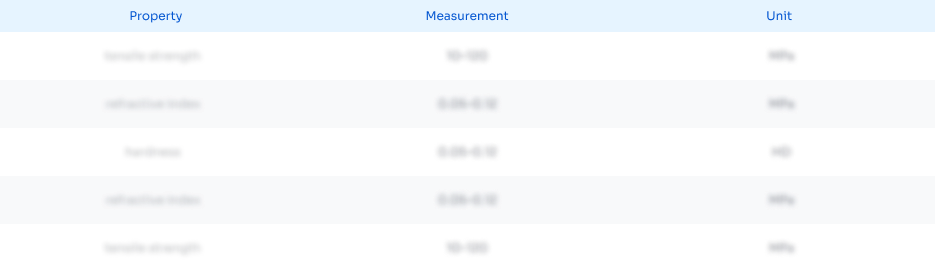
Abstract
Description
Claims
Application Information
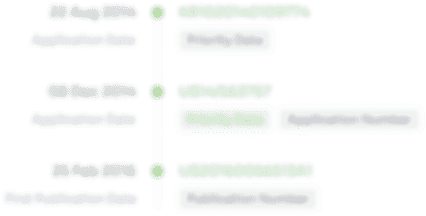
- R&D Engineer
- R&D Manager
- IP Professional
- Industry Leading Data Capabilities
- Powerful AI technology
- Patent DNA Extraction
Browse by: Latest US Patents, China's latest patents, Technical Efficacy Thesaurus, Application Domain, Technology Topic.
© 2024 PatSnap. All rights reserved.Legal|Privacy policy|Modern Slavery Act Transparency Statement|Sitemap