Abnormity detection method based on deep learning in complex environment
An anomaly detection and complex environment technology, applied in image data processing, instrument, character and pattern recognition, etc., can solve the problem of unsatisfactory anomaly detection effect, achieve the effect of optimizing spatio-temporal robustness and reducing false detection rate
- Summary
- Abstract
- Description
- Claims
- Application Information
AI Technical Summary
Problems solved by technology
Method used
Image
Examples
Embodiment
[0041] An anomaly detection method based on deep learning in a complex environment. First, track the trajectory of multiple objects through the long-short-term memory model (LSTM). The temporal and spatial features of objects extracted by the product neural network regression method are input into the LSTM model to track the movement trajectories of multiple objects in a complex environment; after that, the nonlinear temporal and spatial movements between adjacent individuals are captured, and the adjacent individuals are evaluated in the case of irregular movement of multiple objects The dependence of motion trajectories among objects can be predicted to predict the future motion trajectory of objects, so as to complete anomaly detection according to the abnormal probability of individual future motion trajectories. Specifically include the following steps:
[0042] Step 1. Input the spatiotemporal features of the object extracted by the convolutional neural network regressio...
PUM
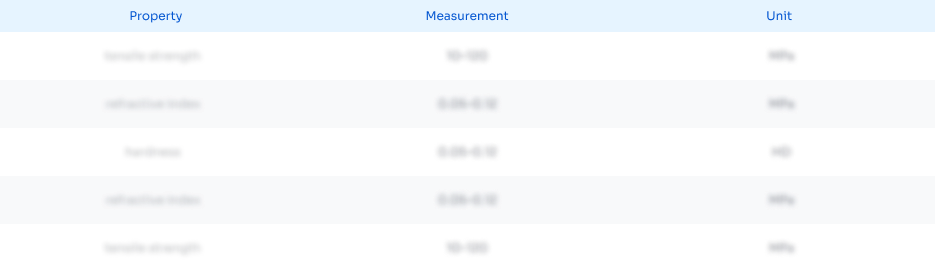
Abstract
Description
Claims
Application Information
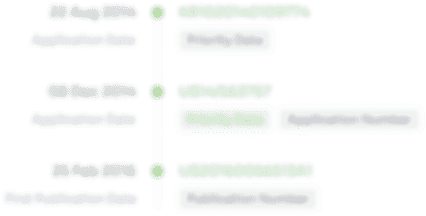
- R&D Engineer
- R&D Manager
- IP Professional
- Industry Leading Data Capabilities
- Powerful AI technology
- Patent DNA Extraction
Browse by: Latest US Patents, China's latest patents, Technical Efficacy Thesaurus, Application Domain, Technology Topic, Popular Technical Reports.
© 2024 PatSnap. All rights reserved.Legal|Privacy policy|Modern Slavery Act Transparency Statement|Sitemap|About US| Contact US: help@patsnap.com