Feature selection method and traditional Chinese medicine main-symptom selection method based on feature groups
A feature selection method and feature group technology, which is applied in the field of pattern recognition and machine learning research, and can solve problems such as difficulties in the study of TCM syndrome differentiation.
- Summary
- Abstract
- Description
- Claims
- Application Information
AI Technical Summary
Problems solved by technology
Method used
Image
Examples
Embodiment 1
[0026] like figure 1 As shown, this embodiment discloses a feature selection method based on feature groups, comprising the following steps:
[0027] Step (1), remove features irrelevant to the label content and features with too small frequency from the original feature set to obtain the feature set to be selected.
[0028] Step (2), using a feature clustering algorithm to cluster each feature in the feature set to be selected to obtain a corresponding feature group.
[0029] For the feature set to be selected D(X 1 ,X 2 ...X n ,Y), where X 1 ,X 2 ...X n is the n-dimensional input feature space, Y is the label, and the feature clustering algorithm is used to classify the input feature space X 1 ,X 2 ...X n Clustering is performed to obtain the corresponding feature groups.
[0030] The key to the Bayesian network with hidden variable learning is the discovery of hidden variables, that is, to determine the number of hidden variables in the network and their positions...
Embodiment 2
[0054] This embodiment is to apply Embodiment 1 to the method of selecting the main symptoms of traditional Chinese medicine, understand the syndrome elements that are the most basic elements of syndrome differentiation in TCM syndrome differentiation and cannot be observed as corresponding hidden variables, use symptoms as characteristics, and syndrome types As a label, embodying syndrome elements in the TCM syndrome differentiation model can make the TCM syndrome differentiation model more consistent with TCM theory.
[0055] like Figure 4 As shown, the feature selection method is used to select the main symptoms of damp-turbidity syndrome. When the feature subset contains the first four feature groups with the highest mutual information with the damp-turbidity syndrome type, the classification accuracy of the classifier reaches the highest, so the features contained in the first four feature groups are selected as the damp-turbidity syndrome type main symptom.
PUM
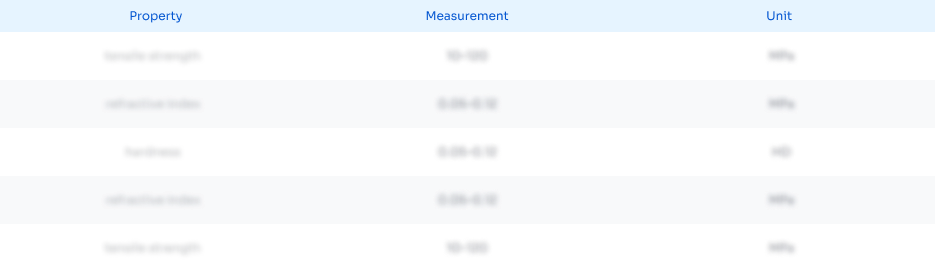
Abstract
Description
Claims
Application Information
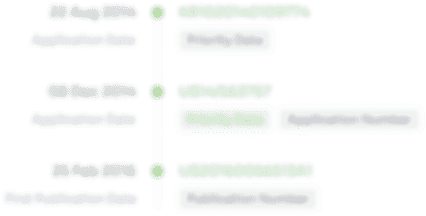
- R&D Engineer
- R&D Manager
- IP Professional
- Industry Leading Data Capabilities
- Powerful AI technology
- Patent DNA Extraction
Browse by: Latest US Patents, China's latest patents, Technical Efficacy Thesaurus, Application Domain, Technology Topic, Popular Technical Reports.
© 2024 PatSnap. All rights reserved.Legal|Privacy policy|Modern Slavery Act Transparency Statement|Sitemap|About US| Contact US: help@patsnap.com