Efficient CNN-CRF network-based retina image segmentation method
An image segmentation and retinal technology, which is applied in the field of medical image processing, can solve problems such as low precision, long processing time, and inability to automatically segment, so as to achieve the effect of ensuring accuracy
- Summary
- Abstract
- Description
- Claims
- Application Information
AI Technical Summary
Problems solved by technology
Method used
Image
Examples
Embodiment Construction
[0023] The method of the present invention first uses the entire retinal image as the input of the full convolutional neural network, and then uses the full convolutional neural network to predict the pixels in the retinal image; according to the output of the full convolutional neural network, uses the conditional random field to The feature image is segmented, and the blood vessel segmentation map is finally obtained through only one forward operation, such as figure 1 shown.
[0024] The method and technical effects of the present invention will be described below through specific examples.
[0025] Step 1: Randomly select 40 retinal images from the international public dataset DRIVE (Digital Retinal Image for Vessel Extraction), 30 of which are used as training samples, and the remaining 10 are used as test images. To solve the problem of insufficient training samples, the present invention expands the number of samples by rotating, flipping, and other operations on each ...
PUM
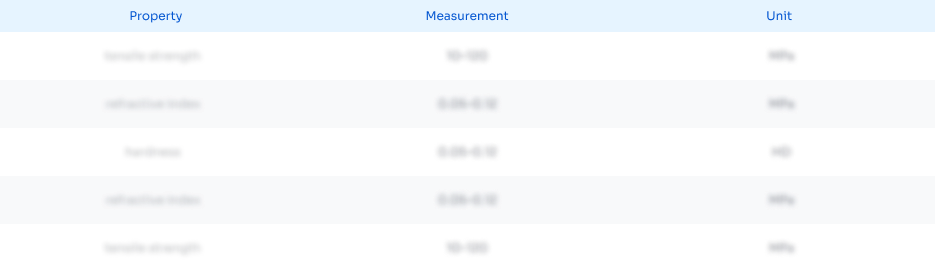
Abstract
Description
Claims
Application Information
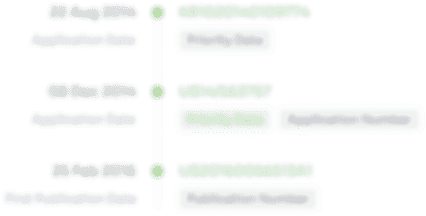
- R&D Engineer
- R&D Manager
- IP Professional
- Industry Leading Data Capabilities
- Powerful AI technology
- Patent DNA Extraction
Browse by: Latest US Patents, China's latest patents, Technical Efficacy Thesaurus, Application Domain, Technology Topic, Popular Technical Reports.
© 2024 PatSnap. All rights reserved.Legal|Privacy policy|Modern Slavery Act Transparency Statement|Sitemap|About US| Contact US: help@patsnap.com