A Spatial Information Learning Method Based on Artificial Neural Network
An artificial neural network and spatial information technology, which is applied in the field of spatial information extraction using deep convolutional neural networks, can solve problems such as reducing neural network dependencies, and achieve robust recognition of invariance
- Summary
- Abstract
- Description
- Claims
- Application Information
AI Technical Summary
Problems solved by technology
Method used
Image
Examples
Embodiment Construction
[0036] The implementation of the method of the present invention will be described in detail below in conjunction with the accompanying drawings.
[0037] A spatial information learning method based on artificial neural network, such as figure 2 As shown, the original image is input into the convolutional neural network (CNN), and the feature map F (feature maps) of the original image is calculated in the high-level space; the filter operation (filter) is performed on the feature map, and the GAP layer (GAP Layer) reduces After dimension processing, multiple feature points (featurepoints) are obtained, a correlation matrix (Correlation matrix) is formed from the feature points, and the structural feature vector CD is obtained through projection (projection) calculation. In the penalty fusion unit (penalty fusion unit), the structural feature vector (CD) and the original feature vector (F) are fused to obtain a full connection output. The specific implementation steps are as ...
PUM
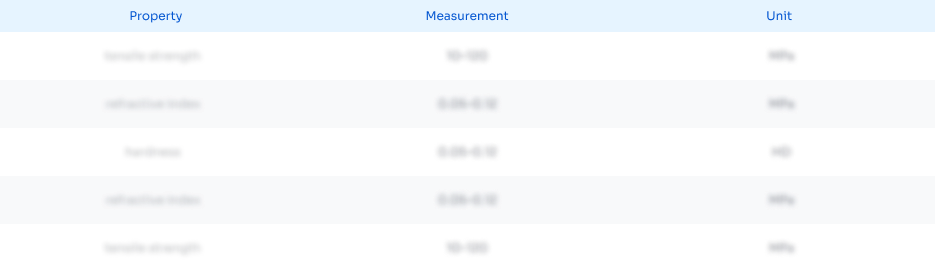
Abstract
Description
Claims
Application Information
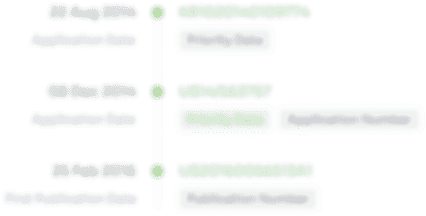
- R&D Engineer
- R&D Manager
- IP Professional
- Industry Leading Data Capabilities
- Powerful AI technology
- Patent DNA Extraction
Browse by: Latest US Patents, China's latest patents, Technical Efficacy Thesaurus, Application Domain, Technology Topic, Popular Technical Reports.
© 2024 PatSnap. All rights reserved.Legal|Privacy policy|Modern Slavery Act Transparency Statement|Sitemap|About US| Contact US: help@patsnap.com