Radar echo extrapolation method based on dynamic convolution neural network
A convolutional neural network and radar echo technology, applied in biological neural network models, neural architectures, radio wave measurement systems, etc., can solve the problems of low utilization of radar data, complex echo changes, and strong echoes
- Summary
- Abstract
- Description
- Claims
- Application Information
AI Technical Summary
Problems solved by technology
Method used
Image
Examples
Embodiment Construction
[0166] The present invention will be further described below in conjunction with the accompanying drawings and embodiments.
[0167] Such as figure 1 Shown, the present invention comprises the following steps:
[0168] Step 1, such as figure 2 As shown, training offline convolutional neural network: input the training image set, perform data preprocessing on the training image set, obtain the training sample set, design the dynamic convolutional neural network structure, and initialize the network training parameters; use the training sample set to train the dynamic volume Productive neural network, the input sequence of ordered images passes through the forward propagation of the dynamic convolutional neural network to obtain a predicted image, calculates the error between the predicted image and the control label, and updates the weight parameters and bias parameters of the network through backpropagation , repeat this process until the training end condition is reached, ...
PUM
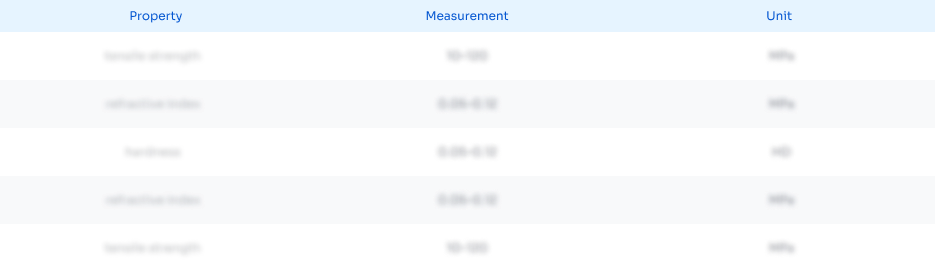
Abstract
Description
Claims
Application Information
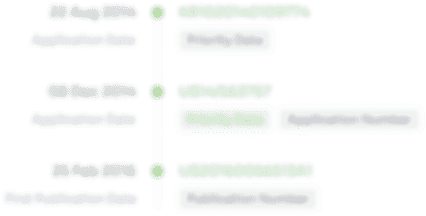
- R&D Engineer
- R&D Manager
- IP Professional
- Industry Leading Data Capabilities
- Powerful AI technology
- Patent DNA Extraction
Browse by: Latest US Patents, China's latest patents, Technical Efficacy Thesaurus, Application Domain, Technology Topic, Popular Technical Reports.
© 2024 PatSnap. All rights reserved.Legal|Privacy policy|Modern Slavery Act Transparency Statement|Sitemap|About US| Contact US: help@patsnap.com