A feature learning model based on adaptive dropout non-negative matrix factorization
A technology of non-negative matrix decomposition and feature learning, which is applied in computing models, machine learning, instruments, etc., can solve problems such as semantic overlap and hidden feature semantic ambiguity, so as to improve feature representation, improve semantic independence, and enhance semantic independence and the effect of distinguishability
- Summary
- Abstract
- Description
- Claims
- Application Information
AI Technical Summary
Problems solved by technology
Method used
Image
Examples
Embodiment 1
[0042]The ADNMF feature learning model provided by the present invention will be exemplified and described in detail below in conjunction with the accompanying drawings.
[0043] The present invention mainly adopts data mining theory and method to analyze data such as text, image and network, in order to ensure the normal operation of the system, in the specific implementation, it is required that the computer platform used is equipped with no less than 8G memory, and the number of CPU cores is not less than 8G. Less than 4 64-bit operating systems with a main frequency not lower than 2.6GHz, Windows 7 and above, and Java1.7 and above must be installed in the software environment.
[0044] Such as figure 2 Shown, the ADNMF model that the present invention provides comprises the following parts that carry out in order:
[0045] 1) Input the data set (text, image or network data), and construct the feature representation matrix of the sample:
[0046] Suppose the input data s...
PUM
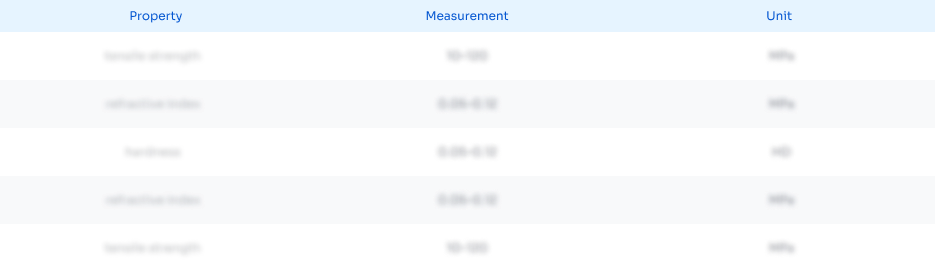
Abstract
Description
Claims
Application Information
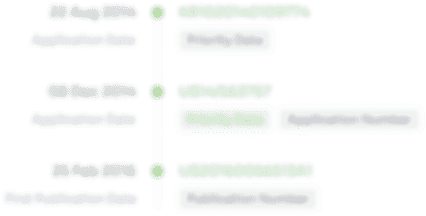
- R&D Engineer
- R&D Manager
- IP Professional
- Industry Leading Data Capabilities
- Powerful AI technology
- Patent DNA Extraction
Browse by: Latest US Patents, China's latest patents, Technical Efficacy Thesaurus, Application Domain, Technology Topic, Popular Technical Reports.
© 2024 PatSnap. All rights reserved.Legal|Privacy policy|Modern Slavery Act Transparency Statement|Sitemap|About US| Contact US: help@patsnap.com